Dan Wagner, CEO and founder of Civis Analytics, discusses the importance of understanding the data journey for nonprofits. He emphasizes the risks of rushing into artificial intelligence without having the fundamentals in place. By highlighting the challenges of automation, Dan offers solutions for nonprofits without dedicated data teams, including collaboration and resource pooling. In this episode we discuss various aspects of data science, including challenges, ethical considerations, and the role of data science in society.
Key Takeaways
- Nonprofits should avoid rushing into artificial intelligence without having the fundamentals in place.
- A use case focus is crucial for successful data journeys, where nonprofits identify specific problems related to revenue or cost and work backward to find the necessary data infrastructure and analytics.
- Collaboration and resource pooling can be beneficial for nonprofits without dedicated data teams. Data science faces challenges such as data quality, bias, and interpretability.
- The future of data science lies in advancements in technology, interdisciplinary collaboration, and ethical frameworks.
- The future of data science lies in advancements in technology, interdisciplinary collaboration, and ethical frameworks.
Chapters
00:00 Introduction
01:24 The Data Journey
06:10 The Risks of Rushing to AI
11:25 Use Case Focus
19:14 Moving into Automation
30:59 Challenges for Nonprofits without Data Teams
36:44 Collaboration and Pooling Resources
10:00 Challenges in Data Science
20:00 Ethical Considerations in Data Science
30:00 The Role of Data Science in Society
40:00 The Future of Data Science
What you can do:
By understanding and optimizing your data journey, you can empower your nonprofit to make data-driven decisions that maximize impact. Focus on specific use cases to identify the most valuable and feasible opportunities for improvement. Embrace collaboration and resource pooling to overcome data challenges, and ensure a strong foundation before diving into advanced technologies like AI.
Resources:
Read an auto-generated transcript of the Episode:
Alexandra Mannerings (00:17)
Today we are going to talk with Dan Wagner from a Civis Analytics and.
Today we are going to talk with Dan Wagner from Civis Analytics, born out of the first Obama campaign and finding the answers to really driving how to help Obama reach his audience and ultimately get elected. We talk today about how we can bring that arc of a journey in creating meaningful, actionable, driving analytics to nonprofits, no matter what size you are.
And Dan is going to bring an outline of this journey and help us understand how we can get started effectively, how we ask the right questions from the beginning. And that sets us up to really progress along this journey in a meaningful, sustainable
Alexandra Mannerings (01:09)
Hello and welcome. I am so excited to be joined today by Dan Wagner, and I’m gonna turn it over to him to introduce himself. So Dan, tell us a little bit about you, what you do, and where you’re from right now.
Dan Wagner (01:20)
Hi, it’s nice to meet you. I’m so glad to be with a fellow nonprofit analytics nerd. We are far and few between with our esoteric abilities and wants. So I’m just honored to be participating in this effort with you. My name is Dan Wagner. I’m the CEO and founder of Civis Analytics. We are a data analytics consulting and infrastructure firm that is highly focused on supporting nonprofits across the board.
Today we support over 50 nonprofits around the world helping enable their data journeys from day one all the way to Artificial intelligence and I’m really excited to have the opportunity to speak to you and your listeners
Alexandra Mannerings (01:56)
Wonderful, we’re very excited to have you. And yes, dated nerds unite, we have to stick together. Fight the good fight. So one of the things that I think is really impressive about Civis and what you do is walking that whole journey. And I wanted to start a little bit and talk about what that journey actually looks like and help someone who is listening orient themselves on where they might be on that spectrum of getting started to artificial intelligence.
Dan Wagner (02:01)
We have to stick together.
Yeah, so I think that the context of the moment is important and that there’s this kind of inflection point in data as people think about, I have a bunch of data, but there’s this tone and trend around artificial intelligence. And while that’s really good, there’s a lot of risk today in artificial intelligence, either from self-initiated wants,
to pressures from executives teams, pressures from boards, to move very quickly to AI enablement or gen AI development within a nonprofit. And as we saw with rushing to big data 10 years ago, or rushing to data science five years ago, there’s a lot of risk in overhype and adopting AI when nonprofits don’t have a lot of the fundamentals in place.
or the use cases or the teams or the problem set to be investing in AI right now. And so there’s this hype and desire and pressure to be moving towards AI, frankly, when people aren’t ready. And if people move to that before they have a lot of fundamentals, they’re at serious risk of repeating some of the failures of the past, like happened with big data, like happened with data science.
And the consequences of that can be more lost money, more burnout, more frustration, which frankly is where a lot of nonprofits are today and their data investments. And so understanding that data journey from day one in terms of what are the investments that people need to make to anchor into the fundamentals to have a successful data program from day one to day 90.
as the key ingredient to getting to artificial intelligence enablement for predictive fundraising for running more efficient programs across the board all the way from hunger to international aid. And articulating that journey is really important because there’s a lot of risk and hype and starting with the end today that can cause the same type of burnout, budget loss and program failure that a lot of nonprofits are increasing today. So, I just kind of want to set up that context
Alexandra Mannerings (04:03)
Mm-hmm.
Mm-hmm.
Dan Wagner (04:25)
And there’s a lot of risk in doing it wrong. And understanding the steps of that journey, both with technology and expertise, is more important than ever so that people can create something that is really good and not repeat some of the, mistakes of the past that have caused so much burnout and frustration at a lot of big nonprofits today.
we want to get through the steps, but I wanted to just kind of back up because that context is so important today as nonprofits are thinking about where they want to direct their capital and time.
Alexandra Mannerings (04:57)
And I think that’s actually a really great place to start before we even talk about that journey is laying the why. Why do we care about the journey? Why can’t we just set out and do whatever we’re going to do and end up wherever we’re going to get up and up? Why do we have to really think about actually identifying where we are and what those appropriate next steps or actions would be? And so I think that context is really important, that idea of that we really do need to know.
what we’re doing now and if we’re doing it effectively and safely before we try to bring something else in that may not be appropriate for where we are on the journey. It doesn’t make us bad or incomplete. We just want to make sure that we’re like right sizing the activities and the expectations and the investments and the activities that we are doing at that point to make sure.
Dan Wagner (05:37)
So what we hear from nonprofits often on day one, and a lot of people are in different stages of this journey. Some of them are on day one, and some of them are truthfully embedding artificial intelligence or instrumenting that into their workflows, either for predictive fundraising, for how they distribute their resources in Sub-Saharan Africa, et cetera, all that way. But frankly, many nonprofits are at day one.
And we hear the same type of quotes from them. My systems don’t talk to each other. I’ve made a huge investment in my Salesforce implementation or my CRM, and yet I don’t have basic and dependable reporting. I know, for example, that my direct response yields are falling, in many cases precipitously. And if I forecast that out two to three years, I’m going to be in real trouble from a budgetary
And I know I need to replace them with online sustainers, but I can’t do that right now because my database is a mess. And finally is I’m really frustrated with everything, often because I rely on my data team, who I’m slamming with requests and often isn’t getting back to me because I know they’re overwhelmed, and I don’t have budget to give them more resources. So I’m caught in this vice. And so the question is, first off, is what are the principles?
designing this journey from how do I start to where do I end? And then what are the steps to get there? And then what are the key ingredients in doing it? And kind of going back to our theme at the beginning is what are the risks and pitfalls that first of all, people want to avoid along the way that might be hype or might be instinct. And the first risk that we hear from folks is investing in the trend before they think about the problem.
People often want to rush to invest in data infrastructure because they think once they invest in data structure, kind of field of dreams, that I might get to an answer before they have a problem. And often there’s under investment in very basic things like data quality. And analytics is so dependable, or sorry, is so dependent on things like data quality, which is super boring, but you get it, that it becomes a pitfall.
And so there’s a few principles in beginning a successful data journey at a nonprofit. The most important thing by far is using a use case focus as opposed to a process focus. A use case focus means on day one and every step of your journey, you’re not asking, what is the thing I need to do? You’re asking, what is the problem that I need to be fixing and then working back from that problem? For example, on day one.
Many people say, I need to invest in centralizing my data and get it all into one place, et cetera. That’s not the right way to think about it. The right way to think about it is, for example, my direct response yields have been falling over the past two years. With whom are those direct response yields falling, and why? In order to solve that problem, you’re going to have to centralize some of that data.
you’re going to have to have some type of reporting and analytics. But if you begin with that one narrow problem, that will direct you in what is some of the data centralization exercise that you need to do. What type of reporting do I need to build? And most importantly, that will help create credibility for a data operation, which is often where things fail. And it will create some type of revenue return that will reinforce.
budgeting priorities, and predictive value. There’s many other kind of day one problems that people can focus on. The collapse in direct response yields is one of the most important. We have a very smart museum customer, and that museum customer has another basic question. And that basic question is, I’m spending lots of money on events, and they want to understand whether or not those events are creating top of funnel visitors.
Alexandra Mannerings (09:05)
Mm-hmm.
Mm-hmm.
Dan Wagner (09:19)
Or it’s just the same people coming through the door because they love wine and cheese, right? And for a museum today where visitorship has declined, that’s really an existential question, is I’m spending a lot of money on this tactic, same people, new people. And in order to solve that problem, they have to be able to centralize their data, run identity resolution between those new people and their legacy database, and understand what percent of those attendees are new.
Alexandra Mannerings (09:22)
Great.
Mm-hmm.
Dan Wagner (09:46)
versus old and how valuable those people are. But again, starting with that use case, which is typically connected to revenue or cost, and then saying, what is the analytics or the data centralization and analytics exercise I need to go through to complete that use case over the beginning and set in place the tools and the process that I need to, to create
a revenue or cost return, which is so essential right now in limited budget environments and the credibility that people need to be successful. And so when we talk to nonprofits, often what we hear is, my systems don’t talk to each other. I spent gobs of money on Salesforce. My data team is burned out. And so the instinct is I have to centralize my data and do all this stuff. And we say you need to invert it.
Alexandra Mannerings (10:36)
Mm-hmm.
Dan Wagner (10:38)
And you need to say, what is the most important problem that you need to solve that is attached to revenue or cost? And what are the ingredients that you need to solve in terms of technology and people to get there? Our focus, obviously, is we provide analytics infrastructure that can pull data from those different CRMs and identity resolution tools to figure out from an event or a visitor who’s the same person or not to help scale that use case focus over
So that’s the first thing is identification of a primary use case that is attached to revenue or cost efficiency, and then figuring out the people and technology that are really successful in solving that at scale over a three to six month term and start there and ignore everything else.
Alexandra Mannerings (11:21)
Mm-hmm.
I’ve had a lot of people, yes.
Dan Wagner (11:23)
Again, that’s focused on day one. Like there’s a long journey, but the philosophy is use case focus and milestones that are attached to different milestones that are explicitly related to the revenue cycle or cost efficiency.
Alexandra Mannerings (11:39)
And I’ve had that discussion with a number of people. We do like to focus on the why, right? That inversion, I think, is really important. It feels obvious sometimes to say, but it is so often missed that we start with the technology, we start with what’s not working about our systems or whatever it is, but actually saying, no, the first place we start is with that use case. But what I like that you’re adding is not just any use case.
not just a question that you thought up when you were in the shower this morning, but like, no, a use case that’s tied to your resourcing because that specific kind of question will generate immediate changes and benefits for your organization. And so then you can get into some of the more interesting, but perhaps esoteric questions down the line because all of the work that you’re doing to answer that resource-based question is gonna also lay the foundation for those later questions.
Dan Wagner (12:15)
Yes.
Alexandra Mannerings (12:28)
But by focusing on a question related to resources, like you said, you can justify the investment that it’ll take to achieve it, and you can easily measure whether you made a difference.
Dan Wagner (12:40)
Yes. Yeah, that’s right. And even more literal, I think some really good nonprofits are saying it’s resourcing, but it is for revenue or cost. And often those two things go together. But cost, for example, is I’m sending a million pieces of mail or two million pieces of mail, and I think there’s a lot of waste because I’m sending the mail to the same people.
And I want to see whether or not I can get the same return by spending less money. Because often in a nonprofit, you have different imperatives, top line or bottom line imperatives, and just being able to say, I’m contributing revenue or contributing costs, and then make that very explicit in terms of like where you’re focused, because often you have different top line and bottom line imperatives. And then top line is, okay, I need to get more sustainers, right?
Alexandra Mannerings (13:09)
Mm.
Dan Wagner (13:22)
And that’s a priority. I know I have lots of like little, lots of things like revenue, but in terms of sustainers, you want to say like, who is sustaining over time? Why are they sustaining over time? And how do I find more people that look exactly like them? Because there’s going to be a long-term benefit on revenue. And so often there’s a clinical interpretation that can help that. And exactly like you said, is when you’re able to,
Alexandra Mannerings (13:30)
Mm-hmm.
Dan Wagner (13:47)
demonstrate success around that, it creates credibility, executive excitement, and technical process, justification, and this type of workflows that you can start to broaden out into other cases.
Alexandra Mannerings (14:01)
And I like that additional level of specificity around the use case of don’t just pick a general resource question, or worse yet, don’t pick a question that spans multiple things, right? That that’s also a temptation of like, well, we just need to have more resources available. You’re like, okay, well, are you going to do that by increasing what’s coming in the door or by decreasing what’s going out the door? And those are, like you said, different initiatives, they relate to different parts of the nonprofit. And so even getting more specific with your starting use case, that’s a really, really
Dan Wagner (14:11)
Exactly.
Alexandra Mannerings (14:30)
rate focus point.
Dan Wagner (14:31)
Yep.
And what we call the day one success is that helps you create fundamentals on infrastructure, team, and process. Because once you have that use case, which is how I’m contributing to the revenue cycle or the cost cycle, I can say, OK, what type of infrastructure do I need in place to centralize the data to make this recurring?
What is the structure of the team that I need to make this investment recurring? And when I think about the next problems, what is the technical ingredients in terms of removing silos, creating automated reporting? How do I think about identity resolution in terms of mapping?
And then eventually is what is the type of automation that I need to build in to be able to support these use cases not just as one-offs, but on an automated basis, which is going to help create that feedback loop of revenue and cost. So thinking about at different steps, what are these use cases ordered by institutional priority? And then what are the different underlying technical things to do it in terms of infrastructure team?
and process to automation.
Alexandra Mannerings (15:38)
Now, let’s say a nonprofit sits down and they make this set of use cases and they identify, you know, we’ve got four high priority use cases. obviously some of the things like identity, the identity resolution are gonna span all of those, that they’re gonna But there may be elements of then the systems, especially when we start getting to automation, that could really differ across those use cases. Would your recommendation be
just pick the top priority use case and go for that. And then when you’re done with that, do your second priority use case, even if they have completely sort of different technical pieces that need to be solved. Or would you say from the beginning, you should be trying to think about how to do all of those use cases eventually.
Dan Wagner (16:18)
Yeah, that’s a great question. Maybe this is too much detail. But the way that we often think about use case prioritization or recommend that people think about use case prioritization using our tools is you have value and feasibility or level of work. Value is what is the contribution to revenue and cost efficiency, if you’re taking it completely literally.
And second is how much work is this going to take to get done? The easiest thing is I can get all the data from one CRM, then it’s like, OK, great. That’s relatively feasible. If it’s I have to sync the data from four different CRMs and match it up and deal with lots of people, and I’m a data team, so I don’t like to deal with lots of people, that’s a lot of work.
through an implementation process, how are people indexing those different problems based on resource efficiency, either cost revenue, and then what is the feasibility of getting that done? And then as you mentioned, the sequencing of that is really important because, for example, if I do it in, I integrate one system and I can get to that next problem with that same data that I’ve centralized, that’s a lot easier.
Alexandra Mannerings (17:22)
Mm-hmm.
Dan Wagner (17:22)
than say, okay, I’m gonna add three more systems and figure out a new problem. And so the sequencing of how you do that based on what data you’ve centralized or not is going to very much impact the level of work for your next incremental problem.
Alexandra Mannerings (17:31)
Mm-hmm.
Right, so the idea is that you kind of backed up and said, well, hang on, how have you prioritized those use cases? Rather than, you know, I sort of shortcut it and said, oh, priority in terms of what’s most important to us or our most pressing question, you said, actually, it needs to be prioritized along two axes, right? Level of work and then the value that you’re going to get. So that makes a lot of sense. And then by using that as your way of prioritization, now you’re going to actually sort of naturally set yourself up to build towards the more and more.
Dan Wagner (17:39)
Yeah.
Yes, that’s right.
Alexandra Mannerings (18:02)
complicated issues and challenges.
Dan Wagner (18:04)
Yeah, that’s exactly right. And then there’s this unknown, see, of X, Y, Z. And X axis, Y axis, Z axis, X axis is value, Y axis is level of effort, and then Z axis is, is there somebody on the executive team who’s really gonna do it? Like, are they gonna, sponsorship is such like a horrible, sponsorship is such a horrible corporate term, but I’m not sure there’s another term, but.
Alexandra Mannerings (18:20)
Mmm.
Dan Wagner (18:26)
somebody who’s gonna be first through the wall on saying to the rest of the executive team, look this is gonna be annoying. And the end of this process is somebody’s job is going to change and somebody might look bad because they’ve been wasting lots of money but unintentionally, right? And we’re all going to be a team on this because often, not often, but always
Alexandra Mannerings (18:43)
Mm-hmm.
Dan Wagner (18:50)
the result of a project or an exercise that’s looking at resources is going to make somebody look real bad because they’ve been blowing tons of money on something or there’s been lots of lost opportunity. And so you need that person on the executive team who’s working with the data principal to say, hey, look, we’re going to be going through this exercise. But at the end of this, I’m going to be pushing to get all the stuff right.
There’s gonna be data access issues, there’s gonna be personalities and like, hey, I’m gonna make this happen. And like, if you’re not on board, we’re gonna have problems. And at the end of this, like, it’s gonna change how we make decisions and like, get on board and do that like as nicely as possible. But that type of sponsorship is really important for making it real. And you can be as abstract about value, abstract about level of effort.
But if you don’t have somebody who’s on board with the outcome and on board with the institutional blocking and tackling, it’s going to be real hard to get something done.
Alexandra Mannerings (19:53)
I’m so glad you added Axis Z because that is probably where I see the projects I work with fail the most because at the end of the day, the technical challenges are surmountable. You can get over them. It might be a little painful. Maybe it costs a little bit more than you thought it would or takes you longer than you thought it would, but you do get over it. But what kills projects like this tends to be that you didn’t have that champion. You didn’t have the executive buy-in. You didn’t have…
Dan Wagner (20:03)
Yep.
Alexandra Mannerings (20:22)
the leadership to carry the team through that uncomfortable period of change, or they let it turn into a competition, like you said, that they let it sour rather than being, no, we’re all on the same side of the table facing the same challenges and it becomes this infighting. But you also have to have it diffused down to where the people who actually on the front line are going to have to change. They have to change what they’re gonna do. They have to change how they do it. And that change is hard.
And so if you don’t have that cultural buy-in, whether it’s top down or whether it’s grassroots, like if it doesn’t exist that we are going to champion this effort, it will just die.
Dan Wagner (20:57)
Yeah, exactly. And I think the conversation that data teams and executive teams miss is this conversation about the data journey where day one is. Or day zero to 90 is identifying something really important and then identifying what is the technology and people to do that.
Phase two is saying, OK, great, I’ve done a lot of that. What is some of the more important analytics that I can do for more challenging problems? And how do I begin to introduce repeatability and infrastructure to solve those types of problems at scale? And step three is, let’s call it this AI holy grail.
But I want to get to a place where my problem solving capacity is more predictive. Or I can say, you’re going to give me a dollar. You’re going to get me a team member. And I’m going to be able to, with some level of confidence, tell you what’s going to come out of the machine. And that’s those three phases. Day one, orientation and fundamentals. Phase two is how I’m thinking about
solving problems with automation and baseline analytics. And then phase three, let’s call it like the fun AI, but predictive fundraising and predictive resourcing that is enabled by this type of, you know, big, I wouldn’t say big, but analytics infrastructure and then really smart people around it. And then participating in that conversation to say, these are the different steps of the journey. What are the problems and what are most important?
What is the connection to revenue cycle or cost? And then what are the different inputs that I’m gonna need to do it in terms of infrastructure and people? And how do we do this on time as a team, dealing with some of the challenges that I might have and some of the sunk cost, frankly, in our CRM environment that everybody feels dumb about, but like, hey, all we gotta do it.
Alexandra Mannerings (22:45)
Do you have a good use case example of somebody you’ve worked with who has done that sort of first phase journey where they identified a good use case, they executed on it, and they got to actually see the results that came back.
Dan Wagner (23:00)
Yeah, I think League of Conservation Voters has been one of our most successful customers who was able to say, you know, gloriously, over several years of working with CIVIS, I was able to improve my fundraising by 140%. And you know, we don’t take credit for that. But our contribution was we were able to provide core analytics infrastructure that helped them.
achieve that data journey at much faster speed and much higher scale. And so instead of doing something over five years, they could do it in two years, and they could do it with far fewer headcount. And by demonstrating repeated success to their executive team, that was able to say, okay, got it. Like, you’re making me money, I’m going to resource it, and this is great. An example of that would be how their analytics leader, this is Amanda.
was able to say, look, we’re at this journey. Step one is we just need to know what is going on in terms of our fundraising outcomes by channel because we have lots of channel, lots of channels, and we’re resourcing them in different ways. And so I just need to see what’s coming out of email, out of direct response, out of text, out of whatever, and just get a report at a regular basis.
that says, what is the outcome from what we’re doing today? And because they didn’t know that, it led to very confusing budgeting. And so she’s just like, I need, we need better prioritization around budgeting, understanding where money is coming and where there might be overlap between different people. Prioritization around more strategic budgeting and the input of that is centralizing lots of data.
and understanding where is money coming from, how much is coming from, what types of people, and then what is the overlap between them. You know, that’s a several month problem. But when she and her team were able to overcome that problem and Sivis provided the infrastructure to help her do that at scale and then some smart people, she was then able to go to her executives and be like, guess what? You have a report that updates regularly.
that tells you how much you’re getting by channel, from whom that’s coming, and where the overlap is. Isn’t this amazing? And they were just like, oh my God, like, you know, heads blown in terms of something that they’ve been looking for so many years. And then once she had that in place, she was able to go to step two and say, look, when we project out two years, we see that our direct response yields are going down.
unavoidable. And if you say right now, I’m netting $75 million in direct response of revenue minus cost. And if I do two years out and I just look at churn rates by people who are aging, that net revenue is going to go from $75 million to $50 million. Oh, God, how do I make that up? And the answer many nonprofits, I have to make that up through recurring sustaining donors. And so who are those sustainers right now?
Who’s coming in through the door? And how can I find more people that look exactly like them? What is the math for finding new people like them? And she figured out that problem. And it took her a long time and took our infrastructure and helped to re-optimize it towards that new problem at scale. So she solved the strategic budgeting problem. And then she looked at and said, we have a two-year revenue crisis.
How do we make up for those donors by creating a new class of donors online? My goodness. Amazing. And then phase three is, how can we do that everywhere? How can we do sustainers, but how can we understand optimization across text, continue direct response, advertising, et cetera, by creating that type of predictability, and math, and automation? And so that was basically her three phases.
And the result of it was a
journey that took a lot less time, but ultimately a level of financial production that was much higher that she would have gotten if she had not had that type of use case focus over her journey and then obviously some of the important infrastructure to help her scale.
Alexandra Mannerings (27:07)
Mm-hmm.
I love that example and we’ve had Amanda on the podcast, which is, she’s an amazing, and she talked a lot about that, that actual creation of that centralized database and things that have to go into it and thinking about it, which I think is a really important piece to look at. Now, one of the interesting, so you talked about pitfalls in phase one, right? That lack of, of buy-in, not focusing on your use case at the beginning with that correct prioritization.
Dan Wagner (27:14)
Yeah, yeah, that’s great.
Alexandra Mannerings (27:32)
across all the axes, which I think is really important. So let’s say we’ve identified our use case, maybe we’ve even made progress on it, but we’re starting to see that return. And we are orienting the work that we’re doing towards that. What I thought is interesting with step two, and I think may be one of the pitfalls, and I’m curious if you would agree with this, would be moving into the automation space. That it’s easier to sometimes build a solution that you piece together and you find an answer once.
but to have something that can continually generate answers over time as inputs change, as the questions might change, even just keeping up with your organizational growth and turnover and churn. So can you speak a little bit, one, if you agree that automation step can be really challenging, and two, what do we do to address?
Dan Wagner (28:16)
Yeah, so this is a tough one. So I say, where do people kind of mess this up? And where I think people mess this up is people, at least in the past, have invested in data lakes where a data lake is I take a lot of data from places and I shove it into one big data lake.
Alexandra Mannerings (28:27)
Mmm.
Dan Wagner (28:32)
And that takes me a year and half a million dollars. And from that, now that I’ve consolidated, I can do stuff. That usually causes a lot of burnout, very frustrated COOs and CFOs because there’s a lot of money spent. And it’s kind of like, you know, what am I getting and how do I scale that? The second pitfall is that a lot of nonprofits
and I want to say this diplomatically, have made really big investments in their Salesforce instances. In many cases, they’re tuned of millions of dollars. And there’s an expectation from a Salesforce investment that I’ll be able to do that stuff. And the fact of the matter is Salesforce is not engineered to do that. It’s not engineered to do cross-channel fundraising optimization or really good.
reporting for non-profits because the technology really isn’t built for non-profits. There is sometimes an expectation that if I do this data consolidation within Salesforce, then I’m going to get this, or I’ve spent so much money on this, can I get the thing? And often they don’t get the thing. The question is, what do you do?
Our focus at Sivis, not making too much of an advertisement, is we try and provide something very lightweight that supports Agile development. And Agile development means for any of these different outcomes, I want to structure a workflow from ingestion, so import, to
ETL, which is basically schema design and restructuring of the data, to either a direct report of, I’ve structured the data and this is a set of views on that data, or a model which is just rank ordering that for prioritization, and then typically shoving that data back into a CRM that says, here are your sustainers that you focus on, or here is your overlap in donations between your channels, etc.
and then beginning to automate ingestion to ETL, to restructuring for reporting or for modeling, and then taking that data and pushing it back to wherever that is, either your views for your report or back into your CRM, which is ultimately where you’re gonna provide donor member communication or maybe it’s just beneficiary programs. But thinking about these problems as workflows,
and how you can scale those workflows from ingestion to report or ingestion to prioritize list production, and what is the technology that’s really good at doing that, right? We provide technology that is really good at doing that, often for nonprofits that are like, you know, this big to this big. If you’re a massive nonprofit.
like, I don’t know, like the World Food Program and you’re allocating billions of dollars in food and sending that all over the world. You know, maybe you want to kind of, and you have higher 50 engineers and think about how to like actually solve that. If you’re a medium-sized organization, League of Conservation, Doctors Without Borders, Greenpeace, some of our customers, you might want something out of the box in order to think about what those problems and how you build those workflows.
Alexandra Mannerings (31:39)
Mm-hmm.
Dan Wagner (31:54)
And that’s how we think about supporting them, instrumenting different workflows against use cases, making them highly repeatable and automated over time.
Alexandra Mannerings (32:04)
I think that idea of agility isn’t critical to thinking about how to get to that automation piece because you talked about the pitfall being investing very heavily in a system that in the end doesn’t actually solve the workflows that link to your use cases, right? Which I think again is where we get in trouble because in phase one we approached the problem on its head instead of orienting it correctly towards that use case, which then makes those workflows pretty clear.
Dan Wagner (32:18)
Yeah, that’s right.
Alexandra Mannerings (32:31)
because you can say, this is what I’m trying to do, here’s where I’m starting, I need to just fill in the gaps and the steps along the way to get there. One of the things I’m curious about with that automation, you mentioned for medium-sized organizations, they can sort of have something out of the box. You’ve mentioned a couple of times with like data teams burned out and things, but I work with a lot of nonprofits that don’t have a data team. They just don’t.
and they might be a small rural hospital and like their data person is actually their director of quality because she’s stuck reporting all these things and so she’s become a de facto data person even though that’s not her training and not what she wants to do. And so I’m curious when you’re speaking to those kinds of organizations, right? Where they really don’t have internal teams to be doing data, how should they be thinking about, you know, I think they can get the use case piece and I think they may even come up and I’ve seen it happen where they can kind of build these
but this is where they get stuck, right? They build a solution where they are copying and pasting something into an Excel. They’re doing some summations, right? Exporting it to this thing, and they copy and paste it into an email and send it to their boss. And like, that’s their process to get the insight they need. So that automation step does just still catch them because so much of it is manual work. And so how would you help them think about making that step into automation?
Dan Wagner (33:46)
There’s the non-creative answer and the creative answer. The non-creative answer is just do what you can do. If you don’t have lots of money, then you’re kind of constrained. Not lots of money. If you don’t have a budget to acquire SAS infrastructure to do whatever, then you’re going to have to have somebody who’s.
Alexandra Mannerings (33:53)
Great.
Mm-hmm.
Dan Wagner (34:05)
prioritizing what are those different problems, and maybe they’re doing some exports in Excel or whatever. Sometimes hiring, let me back up. I think the way that people should think about budgeting these is not just against cost, but over two to three year revenue and cost return. And so they may be under spending on this because they’re saying, look, there’s going to hit.
there’s going to be a one year hit on operating budget that’s really going to suck. And that may be true. And so they need to assess what is the time horizon over maybe two to three years that they should be budgeting against? And maybe the answer is they should be spending more money because there’s going to be a meaningful impact on cost and or revenue. And so maybe that baseline might help them reproject what their investments are.
But the core principle, I think, is once you understand that, you’re just going to need to stage it out. And what can I reasonably do in a period of time? And maybe I should hire some consultant to say, look, I have these use cases. I just need a really basic report. And that really basic report is take it out of Salesforce, get into one place, and then create the five metrics that I really care about. And that’s going to take three months. But that’s what I can do.
And if I don’t get all the fancy stuff, I’m still going to accomplish a pretty huge objective. The other thing, something that some of our customers have done is if you’re working in an organization, with a parent organization and lots of affiliates, is can you work with other affiliates that have the same problem and the same systems? And often they do. They’re chaptered and say, look, I’m here. Are there other two, three, four organizations that are
pretty much trying to do the exact same thing. And can I think about pooling, so instead of paying a million dollars out of my budget, which is insane, can I work with them and provide a vendor or infrastructure provider that can say, can you do the same thing across us because there’s so much efficiency in doing it? And that would be a little bit larger. So I don’t have the perfect response, but I would say,
Think about budgeting against two to three year ROI, especially if you figure that you have a lot of cost waste. Two is you can only accomplish your journey based on who you are and what you have. And three is if you’re an affiliate, who’s there are lots of other people that are trying to do the exact same thing you’re doing, with the exact same data, with the exact same outcomes, can you think about?
with those for tools or partners or whatever, that can help solve that with some type of efficiency and scale that would reduce your cost commitment.
Alexandra Mannerings (37:04)
I think all three of those are fabulous recommendations. And they tie in and build on what you’ve already said in that idea of reorienting the way that we think about the budget. Because again, if you started with a use case related to either cost or revenue, then you’re gonna be able to say, I think I can have this kind of ROI. We just have to right size the expectation of when that’s gonna happen. And I like that two to three year timeline because I do think that that’s realistic. That idea of doing what you can as well ties in
Dan Wagner (37:23)
There you go.
Yeah, yeah.
Alexandra Mannerings (37:34)
to the original discussion we had about prioritization of the use cases, which is you just have to orient the value that you’ll get versus the workload because if it’s what you as an individual contributor can do, then you just have the scale on the Y axis represent what you can do, not what necessarily the whole organization or the team or whatever it is. And that collaboration, I think that’s one that I have just been so enamored with that idea of how do we bring organizations with shared data needs together.
and either you share a higher, right, you can altogether hire one data person who works for all of you, or find a way to bring in some outside help who becomes a long-term partner across all of you. I think that model has so much for it, and you start to strengthen the collaborations and discussions that you can have around what you’re doing with data, and how you’re tackling those sorts of challenges even as the results start to come in from that work. So.
Dan Wagner (38:27)
Yeah, we’re working with one organization, and they have 15 to 20 affiliates. All of them are small, but basically they now have a network of them. And we’re able to create efficiencies because their CRMs are all the same. And so we can just script, okay.
you have this CRM, you have this CRM, you have this CRM. It’s all the same. And so we can write, frankly, an import script that can pull that data from all of them and doing it from one, doing it from 15 is not that much work. Sorry, it’s not that much additional work because the systems are identical. And one, I design it for one, and then getting it from two to 15 isn’t that different.
because the systems are identical. And so we’re able to provide that efficiency to support all of them versus just one of them because that there’s replicability over the technical workflows that we can provide for one versus scaling it for 15. I’m not sure we can do that everywhere, but that is a case where…
we have worked with a very smart network of nonprofits that have similar purposes and similar CRMs and data structures to provide it at much lower marginal cost for each of those different nonprofits. And so I do think nonprofits who are thinking about pooling where they have similarities and can, you know, hire partners or share staff.
Alexandra Mannerings (39:58)
Mm-hmm.
Dan Wagner (40:10)
because they can’t hire a person full time and they can’t hire a vendor to support them. But if there’s similarities between them and other organizations, how can they think about responsible pooling to get that efficiency? Because there are probably those opportunities with lots of them.
Alexandra Mannerings (40:20)
Mm-hmm.
I love it. This has been an amazing conversation. I love such the practical approach and orienting how we actually take on what it’s the whole eating the elephant, right? We can break it down into these real actionable steps. If you were going to leave with one action that somebody could take in the next week that would move them just that inch farther along on their analytic journey, what would be your recommendation for something to do?
Dan Wagner (40:57)
If I was the CMO, I would have a two to three hour meeting with my data team, and I would write X and Y. X is where value equals either cost minimization or projected revenue impact, and Y is amount of effort, and the CMO writes X and the data team writes Y, and you start in Quadrant 4.
It’s gonna be two to three hours, and you’re gonna get to know each other, and you’re gonna get a list of what those things are, and start there.
Alexandra Mannerings (41:27)
And you by nature build the z-axis because you’re having that conversation together. And everybody’s on the same page and saying, yes, we can do that.
Dan Wagner (41:36)
Yeah, exactly. And I think it’s gonna require, a lot of that conversation is gonna require some candor on sunk cost. Because a lot of nonprofits have made really big CRM investments, many of them on Salesforce. And there was an expectation by spending six figures.
Alexandra Mannerings (41:47)
Mm-hmm.
Dan Wagner (42:03)
or even seven figures on a CRM that you would get this. And you’re not gonna get this. No matter how hard you try, those systems aren’t designed for nonprofit analytics. Many of them aren’t designed for nonprofits at all, and especially not designed for nonprofit analytics. And so that candor is just to say,
I’ve made this investment, it’s sunk cost. I’m not gonna see this as incremental above my CRM. This is entirely new. And that is painful for a lot of executives to realize. And just step back and say, I’ve spent six or seven figures on this. It’s not gonna give me what I thought. And it’s not gonna give me these new things. I have to say, what is this next problem?
and how do I solve it with new technology or different people? And have that admittance or that candor to be able to take that? Because if people limit their brain space to say, can I do this with my sunk investments, the answer is no. And if people are anchored into that, it’s going to make progress impossible.
Alexandra Mannerings (43:20)
by letting go. It enables new things as painful as it is.
Dan Wagner (43:21)
You gotta let go, yeah, yeah.
Yes. The first step in solving your problem is admitting that you have a problem.
Alexandra Mannerings (43:31)
Absolutely. Well, thank you so much for your time today. If people want to learn more about you or more about civis, where can we send them?
Dan Wagner (43:37)
They can just go to our website. go to our website and feel free to write in. And we’re happy to have a conversation.
Alexandra Mannerings (43:44)
way.
So that was Dan Wagner from Civis Analytics. I hope you enjoyed our conversation as much as I did. I think that concept of starting with a use case that has tangible impacts on cost or revenue really can break down the barriers of resistance against investing in data, against what can feel like the impossibility of data, because you’re going to see a concrete benefit.
in a meaningful way for your organization. I love the three axes, that idea of prioritizing your data work and where you get really started, where the rubber hits the road, by finding things that are high value in cost reduction or revenue generation, as well as finding the things that have a level of work that is achievable, and making sure you don’t neglect that level of buy-in.
whether it’s executive buy-in that you need, leadership from your team buy-in, or just you to say, hey, this is where I can commit to actually spending my time. So again, don’t forget to check out Civilis Analytics if you would like. If you need that kind of external support on how to get started, my company, Merakanos, loves to do data strategy and help us figure out those really actionable questions that will make a difference. So thank you so much for listening and we will catch you next time.
Good luck and I hope you find the joy in your data along your journey.
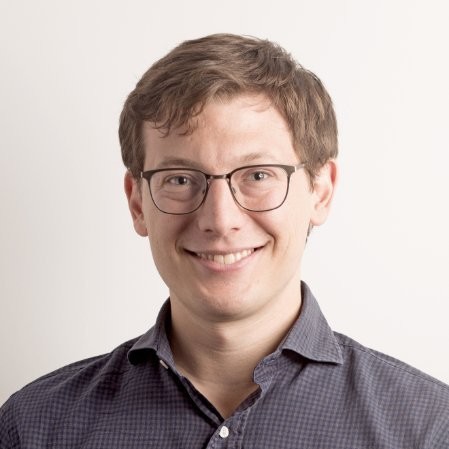
Dan Wagner
Dan Wagner is the CEO and founder of Civis Analytics, a leading data science firm that empowers organizations to use data to solve their most critical problems. With a rich background in data science and analytics, Dan has been instrumental in transforming how businesses and nonprofits leverage data for decision-making and strategy. He is a recognized thought leader in the field, frequently sharing insights on the ethical implications of data science, the future of artificial intelligence, and the importance of robust data infrastructure. His passion for using data to drive social impact continues to shape his work and influence the broader analytics community.
Comments are closed