Great, so you have data! Now how do you make it useful? There is a difference between looking at data and using data. The successful use of data is when an organization is using data to take strategic action related to its goals/direction. Come listen along as Data Bloom co-founders David Mackey and Tim NeCamp discuss their method for making data useful. The four stages they outline are Planning, Collection, Analysis and Reporting and in today’s episode they give lots of helpful information on ways to execute each of these stages and common mistakes they see when going through each one.
You can learn more about David Mackey and Tim NeCamp and their company Data Bloom here.
What You Can Do
Stop for a moment and think about how you (or your team) are addressing each of these four steps. What are you doing by default? What aren’t you doing at all? Who could be part of reviewing your processes? Where can you gain some standardization or remove unnecessary complexity?
Expand to read (auto-generated) episode transcript.
Alexandra
There is a difference between looking at data and using data. And today I’m going to discuss that difference with the co-founders of Data Blue, an organization that is dedicated to helping social good organizations grow their analytic capacity and use data to achieve their mission. We look at the four major phases of actually making data useful to power your social good.
What I love about this conversation with David and Tim is that the stats that they outlined in the framework that we discuss really are usable by any organization of any size at any level of technical capacity. The idea is that you can follow these steps with what you have available right now and some more tweaks and intentionality than necessarily a complete overhaul of how you do things.
Now, you may find in going through these steps and these processes that you want to make some bigger changes. But the point is that you shouldn’t have to make enormous ones. You should be able to develop things that work within your existing processes. So to see how join me for this next episode.
Hello and welcome to Heart, Soul and Data, where we explore the human side of analytics to help amplify the impact of those out to change the world. With me, Alexandra mannarino. I have a special event for today. For the first time, I am joined by two guests. I have David and Tim from Data Blue here. So, Tim, I’ll hand it to you to go ahead and introduce yourself.
Tim NeCamp
Yeah. Thanks for the opportunity to talk on the podcast. Yeah, I really appreciate the time. My name is Tim NeCamp and I am co-founder and head of data Science at Data Blue, and my background is in statistics. I have a Ph.D. in statistics from University of Michigan. And after graduating, David and I started Data Boom to work in the nonprofit space and use our data skills to make the world a better place.
Alexandra
All right, David.
David Mackey
And I’m David Mackey, partner at Data Boom! Co-Founder and I head operations. And my background is actually graphic design, which is what I went to school for and then had a career working in operations and project management. And so I handle a lot of the implementation and logistics and project management for data.
Alexandra
And I feel like you’re partnership is perfect for this podcast because so many people that we speak to really come from such diverse backgrounds and bring their own unique perspectives and skills and experiences to data. And so the fact that, you know, Tim, you have the statistics and the really technical skills and data that you bring project management and graphic design, one, I think it just emphasizes all the different skills that are needed to make data successful, but also that there’s many different ways to get here.
Tim
Yeah, definitely. I think even for ourselves, we knew, you know, we wouldn’t have predicted that we would have been here and we’re in a great spot to be in. But I mean, data boom was an idea maybe like seven years ago. But the fact that we’re actually doing it right now and using our skill sets, which are somewhat different on the service level, but have found a way to combine them to create successful company has been great.
Alexandra
Absolutely. And our two organizations came together. We met because we really do believe in that same thing, the idea of making data available for good. The fact that we can put data to use and drive better and more powerful, more, you know, more rapid change in the world using data is something that I know that we’re all three very, very passionate about.
And that really is what I would love to talk about today is how we go from just maybe having some data around to actually using data effectively using data for good in a way that is impactful. And there’s a difference. And I know that within data boom, you have a framework that spans planning, collection, analysis and reporting, which are the four major domains when we talk about actually operationalizing data for good.
And so today, I would love to walk through those stats and really look at, you know, at a high level what it requires to get there. Before we did those steps, though, I want to just get both of your guys opinions. But what does the successful use of data for good actually look like when we talk about doing that?
What does this look like for a small nonprofit or what do the outcomes? When you have data for good, what does that look like?
Tim
Yeah, it looks like a lot of different things and there’s not it’s not one size fits all, I would say. But I think the first question is like, are you collecting some data? And if you are, that’s a good start. And then are you collecting data that you’re using beyond just the collection? So like a lot of times we’re in non-profits, like, yeah, they’re collecting data and they know it’s important, but after the collection, it stops there.
There’s no like using of the data for any type of understanding their impact or any type of informing their programing. It’s just knowing that they have data is important but not actually using it. So I think actually using it and using it in a way that’s useful to your organization. And a lot of times people think maybe data is just being collected to report out only externally or to like make a case for grants, which is a use, but also if it’s helpful to inform the programing that you’re doing.
Organization helpful to understand your own impact, and playing a key role in strategic planning and meetings with the board meeting internal meetings, Yeah, I think those are all examples of how it’s a success.
Alexandra
That’s a really great point of the idea that it’s not just about using it for required reporting right to an external source, whether that’s your funder or maybe you put it in donor packets or something like that. But the fact that you might collect it and use it for that but not be using it internally, you’re not using it for planning and you’re not using it for self assessment, you’re not using it for learning, then it’s still not really living up to its full potential.
David
Yeah. Echoing Tim, its success is when an organization is using data to take action and then what Tim just said. But then specifically, if those actions are tied to a broader strategy, so to their goals, to their theory of change or to their logic models, that’s when it’s ultimately the success. And I think that goes into kind of that first planning phase that you mentioned is in that phase where making sure that we’re looking at what are your goals and what do you have in line?
And if not really, that’s the first step in all of this is making sure that there’s some star or something that we can always fall back to, to give us that direction.
Alexandra
I always think it’s funny because I’ll have those conversations where people will be like, What data do I want to collect? What should I be collecting, What should I be paying attention to? And I’ll back them up to What’s your organization’s goal? And they’re kind of taken aback often because it’s like, Wait, why do we have to go all the way?
Really, we have to talk about that. I just wanted to know what to put in my table. I’m like, No, because you can’t decide that until you, you know, really where you’re trying to go. So, yeah, let’s talk about that first step of planning When you’re talking about what your goals are as an organization. Can you talk a little bit more about what you need to know about those goals or how you’re going to put those goals in place in a way that relates to then the downstream data decisions?
Tim
Yeah, I mean, I was just following up on what you just said around like aligning goals to what the organization wants to collect as opposed to just like, what data do I need to collect for data purposes. I think what data you need to be collecting really should just come for free from like your strategic plan and logic models.
You know what I mean? It’s the same question as what impact do you want to have in your organization and the data that you’re collecting should be directly aligned with that. It shouldn’t be some extra thing that you’re collecting that doesn’t have any alignment with your goals or strategic plan. It should really be fully aligned, and I think that is usually the place to start is whenever we’re working with organization, maybe they have some data that they’re not using or maybe they don’t collect any data at all, which happens a lot of time.
So, you know, if you’re out there and you don’t have any data being collected, like don’t feel like you’re the odd one out. Like that happens a lot of times. And the question is what data to collect. It’s always like, okay, let’s see what your strategic plan is, your logic model and what impact you’re trying to have. And then we can decide what data will both inform that and help you understand if you’re having the impact you want.
David
Another useful thing that we find in this planning phase is clarifying for people what data is, because sometimes you’ll meet people that say or they think that data is something special or different than what they do themselves in the day to day. And if we sense that’s the case, then we’ll just switch information instead of data and say, What information do you have?
To Tim’s point, some people are not intentionally collecting data, but everyone has data. Like no matter what you’re doing, if you know the names of people you serve or what you’re doing in the most basic capacity, you have data. And I think that we also emphasize that you can take action from that. You don’t need big data, you don’t need to be crunching tons of numbers.
There are ways to get insights and take action from something that is your basic day to day.
Alexandra
I joke that data often feels like a four letter word for a nonprofit, and so I love that idea of saying, Hey, if this feels confusing or you think that somehow data is going to require you to become a computer scientist overnight, well, just talk about information, because that is really an interchangeable thing. I say that I define data as adding kind of information that can be stored and recalled.
So if I can put it somewhere and I can recall it, I can get it back somehow, then it’s data, whether that’s pictures or recordings or notes I wrote on paper or whatever it is. All of that are different types of data and it is easy to forget that those are all things, and it is unlikely that any nonprofit, no matter how small, is out there operating without generating some kind of thing that creates a permanent record of some kind.
Again, whether it’s just notes on your notepad or whether it’s transactions that you didn’t realize you could extract, and that becomes data that it’s there and that maybe it’s not even you have to collect anything new. It’s more about the fact you just have to be more intentional or recognize about what you have that you can already use.
Again. Tim, to your point, there is an alignment with what you’re trying to achieve as an organization.
Tim
And also like, yeah, I think intentional and maybe a little more organized, you know, like what happens a lot of times it’s like maybe you just have these all in just a bunch of like documents saved on a file or something and it’s like, oh, maybe putting some documents into a spreadsheet. Now it’s the same information. It’s just a little bit more organized and you can maybe make some stronger statements now that you have it all in one spot or even like, I mean, I’d go as far to say, like, it’s even in your head, like you have stuff stored and you can recall it in your head.
And maybe it’s a matter of getting it out of your head and like onto a spreadsheet or even onto a document. And just starting to record it outside of your head is the beginning of some type of data collection.
Alexandra
So in planning, we have making sure we know what our overarching goals are. Those North Stars you talked about clarifying what we mean by data or information so that we cast our net broadly enough to find the pieces that we need towards those goals, and that oftentimes it’s not even about needing to collect new stuff, but about organizing it or fine tuning what you already have or getting it from either your head or a system that’s not very usable into a spreadsheet or something where you can start to work with it.
Then I assuming we move from planning into more of that collection design and actual implementation. So can we talk a little bit about that stuff?
Tim
Yeah. The next step, once you have an idea of what type of data you want to collect and yeah, I think the next logical step is figuring out really how to collect it. And this is, I would say like as a statistician and as someone who gets really excited to analyze data, this is the maybe oftentimes, like least enjoyable or not, it’s fun step because it is maybe more of the grunt work.
And it also sometimes and oftentimes is the most time consuming and it’s a lot around just like collecting and organizing to eventually get to the analysis and eventually get to understanding it. But it’s also one of the most critical steps because I would say if this data collection is not thought deeply about, then either. One of two things I think can happen.
One is that you it’s really cumbersome to collect the data that you want, and it becomes this really time consuming task that is just taking up more time than it needs. You know, like there’s a lot of ways to automate processes or collect data in different ways that can be really fast and really easy and kind of work within your workflow.
But if you don’t think about it carefully, then it can take up a lot of time. And then what often happens in that and once it becomes something that takes up too much time and it’s not that it’s just taking a lot of your staff time or time or a lot of people or one whole person whose job is dedicated to it, then oftentimes the data collection goes to the wayside and the data stops being collected.
And you may have set up all this planning and all these ideas about what kind of data you want to collect. But then after three months of collecting it, you stop collecting it and you no longer have the data that you need, and it just becomes a spreadsheet that hasn’t been opened in three years.
David
And so jumping on top of that in that planning phase, where part of that is identifying the processes that organizations have in place. And then for the collection, we really emphasize fitting in these collection methods to the existing processes. Now if we can make those better or more streamlined, that’s key. But really from like the implementation side of things, we’re looking at touchpoints, whether that’s with clients or with data information and then seeing, okay, where can we automate this or how can we make this just easier for the people?
And that’s how you get buy in. It’s because you’re not making it harder. You’re not coming in and just saying, okay, I know you’re used to working in program X, but now you have to do everything and why we really come in and work with what they already have and then that makes it easier. And it’s an easy win because they see, Oh, this actually will make this process much easier on me.
And so from a collection standpoint, a lot of times that’s how we approach those, not just what, but the hassle is just as important.
Alexandra
I love that both of you brought up the human element of data connection. So this idea that it’s not just about the technical collection of data, but you have to make sure that the processes that you have to collect data are not too burdensome on people because then they just won’t do them right. And a perfect quote unquote data collection process that’s not done is not a perfect data collection project.
And so the idea of really saying, how do we engineer this not just to get us the data that we figured out we needed in the planning phase, but to create a process that is bearable for the people who are going to have to execute on it. And David, you mentioned fitting it into existing processes where possible with the idea that you’re minimizing the extra weight you’re putting on people and that you are streamlining how much time new like new learnings have to happen, figuring out new things like, no, keep it with what you already have so that you actually have people willing to continue over a long period of time to maintain data collection because data for this much time, right, is really not helpful. You need it to continue on in order to become part of your strategic plan.
Tim
Yeah, I think just trying to make things more concrete for those of you out there who are wondering what all of these like high level abstract terms are about. I think an example for an organization that we are working with is, you know, they’re just like a community engagement organization. So they just have meetings occasionally throughout the community and trying to bring people together to do some community education.
And that’s really the impact that they’re trying to have is just different. Having different community members and stakeholders attending these meetings, providing some information for them previously, you know, their data collection, they know they needed data was, but it was kind of just all over the place. Like some meetings they would write down just a number. We had 15 people attend.
Sometimes they would write down everybody who attended and what community organization they’re coming from and all this information. Sometimes they didn’t record anything at all just because it was like a lot and they just forgot in the moment. And so I was like thinking through, okay, how can we make this really easy for you? How can we standardize stuff so that way, like multiple meetings, can collect some similar information and you can do aggregation of total number of attendees and total number coming from different counties in the area.
And how can we make it really easy and how can we ensure that data is being collected? And honestly, just doing like a little ten second survey of all the participants in the middle of the meeting, not at the end when people might forget to fill it out or might be ready to leave in the middle of the meetings, have people take 10 seconds, fill it out, have a standardized list of the organizations and community in like counties that they might be coming from.
Have that same, you know, survey be filled out every single time, gives them all the data they need. And it’s standardized every time. And it takes really no additional work on their end. They just have to send out that link in the middle of their PowerPoint. Usually it’s just posted on like a QR code and they’re getting all the data they need.
It’s consistent, it’s standardized, and it takes no additional time. And that’s like a sort of ideal case of thinking deeply about data collection can save time and make sure you get the right data that you want.
Alexandra
And when you’re thinking about standardization, it’s not just, well, we’re going to collect all the data and we have to collect everything we need or everything that we could. You’re using your planning phase to say, what is it that we actually need? And to your point, not just need for funders, but need for our internal planning. And you will have identified those points and then you just make sure that you’re standardizing the collection so that you’re consistently getting those pieces of information that you needed identified in your planning.
Tim
Yeah, exactly. I mean, it’s also a consideration too, of like, yeah, who you collecting the data from and what’s reasonable to collect from them. You know, like it’s not reasonable for everybody to be filling out a three minute survey in the middle of these meetings. It’s going to really break up their flow. It’s going to take away from the programing that they have already thought deeply about and the impact they want to have.
Like, let’s make it quick, let’s make it concise, let’s make it focused on exactly what we will need and use going forward. Yeah.
David
And that can be an iterative process too. You could start off by doing it at the beginning and then realize, Oh, you know what? We’re not getting the late comers or the end and we’re not getting everyone or Hey, we have credit hours for continuing education with this. And that incentivizes people. And so working with an organization to do that, I’d say one other note that comes to mind is it’s really powerful to see when like someone gets it and an organization is surprised that, oh, we can use like the same types of things that we always use, different things come to mind.
But whether it’s the CRM or just forms that they use for everyday internal stuff and they see the potential and we can help them reach that potential, that’s always kind of the light bulb moment is fun.
Alexandra
I agree that iterative piece gets missed a lot. I think a lot of people will put a lot of effort into the planning. They’ll design the how in the collection and figure out, okay, this is how we’re going to do it. Maybe they purchase a new piece of software or technology and they set it all up and then they never come back to change it or say, did our initial assumptions worked at the way we thought we wanted to do it?
They just set it up once and move on. And I think there’s really a missed opportunity for optimizing that process because you’ll take your best guess. Getting started. But if it’s new to you, if you’ve not done it in this way, or if you’ve not standardize it in this way, or you’re collecting new elements, not knowing what you’ll know after doing it for a little bit, you know, you really might miss some of those pieces.
And so giving yourself a space where maybe you just do minimum viable to start and you give yourself that chance to iterate, You don’t just like invest in fully developing it and never coming back, but instead try it in a small way, see what works, modify it, reassess and get it really to the point where it is efficient, lightweight, collects what’s reasonable.
And to your point, if it uses the tools you already have before you go out and think you need something new, what aren’t you taking advantage of that you already have and you could just fine tune it by trying some things and iterating in new ways.
David
And it is helpful to have a partner in that process. But ultimately the goal is that capacity building so that the people in the organization are thinking, Oh, okay, that is the possibility and we can do this, and where else can I apply these concepts that I’ve learned too? And that’s usually what shows kind of a job well done on our end.
Alexandra
Absolutely. And I think having someone to help you get started is really important. But you’re right. The right partner is going to help develop those internal capacities and those internal skills because really everyone has a role to play in data. Virtually everyone in the organization is going to touch it in some way, whether they’re part of the collection, whether they’re part of the maintenance, whether they’re part of the planning, whether they’re one of the users of the outputs of the data.
Nearly everyone is going to touch it. And so if they understand their role and understand how they can contribute to that overall process, you’re going to find that you can build nearly all the capacities that you need within your organization, across the different roles. All right. So we’ve figured out what data elements we might need in alignment with our strategic plan and the goals and where we’re trying to get to.
We’ve developed reasonable, as lightweight as possible optimized data collection processes. Hopefully then we put them in place, we trial them, we tweak them, and then we get to the analysis step. And I think for people who self-identify as non data, people say that sometimes analysis can feel a bit overwhelming. So let’s talk about what analysis actually looks like and what it might take to be successful with it.
Tim
Yeah, just going with the theme, I think analysis looks like a variety of things, depending on what the original goals were, but I often think it’s funny is like a statistician with a lot of formal training and heavy theoretical statistic. X I know a lot of analysis techniques that would be very scary for a lot of people, you know, and they are kind of scary.
I mean, they’re still scary for me, I would say. But even like what I found, especially working in the nonprofit technique or nonprofit space, that a lot of these techniques aren’t really necessary. An analysis can actually be like relatively simple, and that will be maybe the most useful thing that a nonprofit needs. So yes, you could be calculating P values or yes, you could be doing some type of predictive modeling, but really, like the most useful stuff is oftentimes like averages or medians or just segmentation.
And like looking at the specific averages or medians for certain subpopulations or certain demographics. But overall, like analysis doesn’t have to be anything crazy fancy. It can really just be the thing that that will give you the information in the summaries that you need. And so, yeah, I think that the other component too, and another component of analysis that we have found very useful, again, going back to like the simpler side of things is visualization as an analysis technique.
So analysis is really about understanding data, and data visualizations are really some of the most useful tools for understanding that data. So yeah, I think analysis is a scary term, but it can be done in very simple ways and that can provide a lot of really useful information Without getting too complex.
David
I think it’s helpful to keep in mind the point of analysis, which is usually to gain insights. And so knowing that will inform how you go about it. And to Tim’s point, which methods you use. But really what we’re trying to do when we go into the data analysis is figure out what it’s saying, what insights that are relevant, and it’s also helpful to have a hypothesis.
So a lot of times we’re having a conversation with the organization where we’re figuring out what they think or what story they’re they might be trying to tell. And yeah, like going from that point and then helping again, you have to be careful of bias in the analysis, but figuring out what we do to look at what data we got.
I think the other thing is that exploratory analysis is often very helpful and that can be through the visuals, that can be depending on the data that we’re looking at, can be really helpful in seeing what’s going on and helping to get to those insights.
Alexandra
Yeah, I noted that really you could simplify the same way we went from data to information because that was a less scary word that you could go from analysis really to just two steps that are needed to take data into understanding. Right? And it might be as simple as just summing things up, right? So if the point of information you needed was how many people came to our events and what you have in your data is one entry for every visitor or one entry for every ticket sold, then the only analysis step that needed to go from that to how many people came is to sum it up, right, to say, All right, we’re going to just count these up. We’re going to know the total number. I think part of what makes it scary for people who aren’t as comfortable in the analysis space is they think, well, what if I don’t know something, right? What if I’m missing the step that’s actually needed and I just don’t know about it and I don’t know that I need to use it to get from here to there.
And I think that goes back to your earlier point that you can get started with a partner. You can get started with somebody who is comfortable in their space and they can say, Yeah, you’re good. You can keep using these simple techniques that you have and that’s totally fine. Or they’ll point out where you might need a little bit more sophistication in calculating it, and then you can develop some of those internal skills to be able to continue forward.
But complexity does not have to equate to usefulness.
Tim
Yeah, and oftentimes they’re negatively correlated.
Alexandra
Right?
Tim
More complexity is less usefulness. And if your analysis leads you more confused, then you haven’t gained really understanding from it. So yeah, agree. And I think to David’s point, in terms of exploratory data analysis, I think it is all about like the iterative process. You know, like maybe we look at the sums of all the attendees that have attended all our meetings and we’re like, okay, cool, we have this number, it’s kind of useful.
But then that starts your brain thinking a little bit around, like, okay, what about like all the number of attendees in this specific county or all the number of attendees from these types of organizations? And then you, you know, maybe you’re doing a little bit more complexity in your analysis from there, but it’s like, yeah, don’t be afraid to just do something simple and start like having conversations about it.
And then the more complex analysis might come up intuitively and naturally from wanting to dive in further on some basic statistics.
Alexandra
Absolutely. Now, I know we touched on the last step a little bit when we talked about data visualization, but we’re now, you know, we’ve gotten through we’ve transformed our data or information that we’ve been collecting through analysis into that insight we need. We have that last step of reporting. So what do we mean by reporting? What does successful reporting require to get put in place?
Tim
Yeah, and I know I mentioned data visualization and I kind of I would be tempted. I think data visualization is very useful for reporting. But also in terms of the exploratory analysis, it can be useful as an analysis technique. Maybe you’re not making a visualization that’s going to go in some type of final report, but if it’s visualization that helps you understand the data a little bit better, then that can be a part of the exploratory analysis.
But yeah, I think kind of jumping into reporting, I feel like also term that maybe causes people’s eyes to glaze over because they think of a data analysis report and it’s just like maybe a 50 page document with a bunch of graphs that aren’t that useful. But I think reporting I’ve found can look like a lot of different things.
And some of the most useful reporting that we often do, especially in the nonprofit space, is like data interpretation parties where it’s like, okay, we did some analysis, we found some interesting findings, and now we’re going to have not like a presentation where we’re just telling you what those findings are, but we’re going to have a discussion, an open ended discussion where we’re showing off some of this stuff.
And we’re seeing like, you know, we have maybe some interpretation that we’ve come up with based on what we saw, but we’re having the nonprofit and maybe community members also be like, Oh, this is interesting because of this. Or like, Oh, it would also be cool to to see this broken down by race. There’s something like adding in understanding, adding in interpretation, and having this dynamic process to really like seeing what we found from the data.
And so, yes, reporting can often look like a report or some type of PDF that’s sent around, but it also can look like a conversation.
Alexandra
Absolutely.
David
In our process, that’s often part of the analysis or like equitable analysis, because a lot of times for that analysis you’re starting by answering questions or trying to answer questions. And then we’ve found that the more people you have more perspectives that come and look at that they’re asking different questions and then that helps inform that analysis portion.
And that ties into what Tim said in terms of those data parties or being able to have those sessions where you’re gathering that info. And then ultimately that ties to the data reporting. And the last thing we want to produce is a 400 page report that just collects dust on a shelf somewhere. And so a lot of times our reporting is purely focused on the audience for which we’re reporting to and the intention of what they’re trying to convey.
And so it isn’t usually a report, sometimes it is, but a lot of times it is a presentation or infographics or other materials that help convey the main points so that people aren’t going to get lost in the details. And it can just really focus on conveying whatever information we’re trying to convey.
Alexandra
Absolutely. And I think there are two really interesting things. One, you, Tim, were saying that visualization, especially interactive visualization, really probably is more analysis because you’re interacting with the data, you’re diving into different things, you’re exploring and you’re using that to really make sense of the data. Whereas it’s difficult with especially an interactive data visualization to really tell a story because it’s open ended.
You can click and filter and do whatever you want, and by the time it gets to reporting, you’ve done all the analysis you’ve explored, you understand what it is that you want to say, you understand the story you’re telling out of it. And so you want to then sort of home in on that particular story. So David, you mentioned an infographic, so you’re going to take the pieces that you discovered in your data visualization and you’re going to weave it into that infographic story and really maintain that that storyline that is relatable and aligned with the audience that it’s intended for because there may be multiple stories you’re telling, right?
One audience might need one story and another audience might need a different story. And so you’re going to pick those out and create separate reports for them. I love the data interpretation parties. I’ve had several guests mention them. One in particular, Veena. She talks about creating data placemats so that you break data up into these little bite sized chunks and you can lay them out on the table and people can move them around and understand, okay, well, I see this trend over here and I can see this summary over here.
What do I have in my experience or my knowledge that could help us contextualize this or understand that? And that’s where you do really start to move from the raw data to the analysis to actually really understanding it and making it something that is actionable. Do you have any recommendations? Again, it’s one thing to get insight, it’s another to get actionable insight.
So once you’ve gotten to that last bit where we’re reporting, what do we need to be really paying attention to to make sure that we are getting things we can take action on?
Tim
Yeah, that’s a good question. And I would say I would say my initial reaction is to say that like it’s less about the one specific thing that you found in the one specific report that you want to take action on, but more about the reporting processes you have in place. And I think a key part of that is having consistent check ins and consistent understanding of what the newest data is, what the newest reports are, and making sure you’re taking time to like, look through that, look through those reports, have a discussion with your team, with your organization around those findings, and then taking action from there.
So I think, you know, a lot of times maybe it’s just at the end of the year, people are creating a report that they need to report to their board or report to some grant, and they have all these findings. Maybe that would have been useful to see like three or six months before. But if you have processes at your organization to like check in on those on the most recent data and have discussions about it, I think that is what often leads to actions is because you’re setting yourself up and you have the processes in place to to actually understand the data in real time and take some type of action.
You’re not just reporting for reporting sake.
David
I would say that actionable insights are often part of the analysis step in the process in terms of because of that constant communication, because of that analysis where we’re asking questions and we’re convening and meeting and seeing what this actually means, and then reporting is part of that dissemination plan that comes into that. So reporting is often an action that we take based on that analysis.
And like Tim said, whether that is to the funders or to the clientele or the beneficiaries, any number of people that need to have that reporting that can be catered to. But really those actual insights tied to the actual analysis so that it is more real time. And that also ties back to that internal capacity building where once you have a dedicated individual or if you can identify someone who really kind of understand or at least sees the potential, then that goes a long way in helping to make sure that not just they understand what the insights or what actions they can take, but what can their other teams take.
And so the higher up you are, if you’re at a director level or C-suite level in an organization that goes a long way in helping to say that when they see the big picture, they know what insights can be actionable and direct it from there.
Alexandra
Yeah, that makes sense.
Tim
Yeah, I was just going to add on one more thing in terms of like processes. I think like hopefully you have an idea that your your data will provide good insights and actionable insights from the planning phase like that. Hopefully it’s already thought about like I know we’ve collected this data with the intention of it being actionable and you can kind of predict that from the planning phase.
But all that being said, it’s hard to predict exactly what the data will show and what happens once you see the data. And so a part of those processes is like that. What we were talking about before is the iterative process of like, okay, yeah, cool. We got all the number of people that attend our meetings, but we kind of knew that number anyway that wasn’t that useful.
It’s like we really have deeper questions about like who is being represented at these meetings. And maybe we, we did in the planning phase, decide to collect, let’s say, racial or demographic data or like location data. And so missed out on that representativeness that we really wanted to have to inform some of our actions. Boom, we’re going to add that into our actual data collection.
And then next time, you know, maybe in three months, once we start collecting some data, we now have some of the actionable insights that we wanted to have. But it’s okay if maybe the first time you collected and reported on and analyze reporting on data, it wasn’t that actionable, but it might cause you to ask questions that can be answered with data and can be acted upon.
And so that’s a very powerful first step. Maybe in the second iteration is when you have the really actionable insights. But we are taking these steps in that direction.
Alexandra
It’s the perfect conclusion because we’ve come full circle and it’s that same thing that you don’t just put it in place once and leave it alone. They feed and you create this positive cycle. I say, it’s not a circle, it’s a spiral that you come back, but you’re higher than when you were before and you can keep spiraling your way up.
But it requires continually revisiting each of the steps. Stopped one and done.
David
Yeah.
Alexandra
Excellent. Well, thank you both so much for your time today. If people were interested in following up with you or learning more about data bloom, where could they go?
David
Our Web site is probably the easiest that’s: databloom.com. And from there you can see kind of branch out, see what kind of work we do. Learn a little bit more about myself and Saadia, who works for us based in Ann Arbor.
Alexandra
Excellent. Well, thank you both so much for your time today. It was such a pleasure to talk with you.
Tim
Yeah. Thank you. Thank you. Fun to chat.
Alexandra
That was David Mackey and Tim NeCamp from Data Bloom. We talked about the four phases that they work through to really ensure that the data you have and you create are useful for driving at the most change you can with your organization. So step one is planning and this really isn’t necessarily about planning all of the elements of your data, but rather planning based on what your goals are.
So getting clear about what you’re trying to achieve, what those North Stars are that are going to guide you, and how you can have the data that you need to support the actions outlined in your strategic plan. So Tim points out that really your data element should just naturally arise from the outlines of your strategic plan and then converting your strategic plan to those particular tactical actions you’ll need to take.
Then once you’ve outlined in the planning what information you really need to know in order to be successful all the time, not just your year end report or presenting to your board, then it’s time to say how do we actually collect that information? And it may be that those processes are already in place and it’s just a matter of refining them or getting more consistent.
But if you do need to create new collection methods, you want to make sure, as David points out, that those new collection methods really align with what you’re already doing with the steps that you already take to do the work that you do on a daily basis with the systems that you’ve already got in place, there is a need to make sure that you are standardizing the critical elements that are feeding your strategic plan and your initiatives and your learning culture.
But the standardization should be less of a burden and more about just being consistent in getting the information that you need all the time. Don’t be afraid for this to be an iterative process. Don’t make this a huge thing where you’ve tried it once and then you can never change it, make it small, make it quick and nimble so that you can try something and modify it if needed.
As you go through this collection process, once you finally have data, we move into that analysis phase as Tim said, that really complexity is oftentimes not only not supportive of usefulness, it can be counter to being useful. So this isn’t about making super fancy statistical methods, and this comes from a statistician. It’s about just taking that information and turning it into the insights that you need and don’t forget, visualization is a really powerful analysis tool to help make sense of that data.
Lastly, we have that reporting stuff, and David helped clarify this fact that reporting is it necessarily about when you take your action, it’s about disseminating that information. It’s sharing that information with people who might need it. So oftentimes the actions may come out of that analysis step and the reporting is more about maybe informing key stakeholders or driving that information around the organization to the people who might need it.
And there you have it, the four main activities of actually making sure your data are useful. If you would like a partner to walk along this journey for you. I highly recommend that you do check out data Bloom at the data Bloomberg.com. Great team, great support there for you in walking through this whole process. Thank you so much for joining me today.
This has been heart, soul and data with your host me Alexander Manfred’s. You have been listening to heart, soul and data. This podcast is brought to you by Merakinos. It’s an analytics education, consulting and data services company devoted to helping nonprofits and social enterprises amplify their impact and thrive through data. You can learn more at merakinos.com.
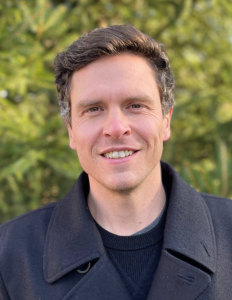
David Mackey
David is passionate about working with mission-driven organizations to achieve impact. He has 14 years of professional experience in design, operations, and project management. As a project manager, David guides organizations, teams, and individuals towards a shared goal. He will ensure our work aligns directly with your mission and theory of change. His design experience allows him to think creatively to solve problems with data. This design-thinking has informed David’s belief that everything must be done with purpose. His operations background has shown him how to use data and technology to improve efficiency and implement successful initiatives throughout an organization. David has seen the value of data to inform decision-making throughout his career and is excited that Data Bloom allows him to partner with those making the most impact in the world.
David has a B.S. in Graphic Design from the College of Design, Architecture, Art & Planning at the University of Cincinnati.
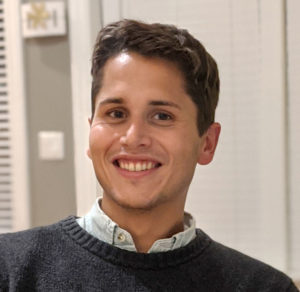
Tim NeCamp
Tim has spent his adult life seeking out ways to combine his interests in mathematics with his desire to make the world a better place. Tim first fell in love with community development and working with vulnerable populations to create meaningful change through Teach For America as a middle school mathematics teacher in a high-needs school district. While in graduate school, Tim used his statistical knowledge to impact his community by volunteering for Statistics in the Community (STATCOM), an organization that provides free statistical and data consulting for local nonprofits. As a member, project leader, and eventually the president of STATCOM, Tim saw how data thinking and data usage could help community organizations achieve their goals. Tim is excited that Data Bloom allows him to dedicate his time, energy, and resources to continue this work.
Tim has a B.S. in Mathematics from The Ohio State University, a M. Ed. from University of Nevada Las Vegas, and a Ph.D. in Statistics from the University of Michigan.
Comments are closed