In this episode, SaaS CFO, data nerd, and Excel fanatic Matt Brattin joins Alexandra to explore the key elements of successful data management. They emphasize the importance of consistency and integrity in data collection, as well as the need for data to be accurate and usable for its intended purpose. The conversation covers practical tips for cleaning and transforming data using Excel, highlighting its versatility as a beginner-friendly tool for data analytics. They also provide valuable resources for learning Excel and improving data literacy. This episode serves as a concise guide for those interested in harnessing the power of data through efficient data cleaning and Excel proficiency.
Resources:
Click to read the auto-generated transcript of today’s episode
Alexandra: Well, thank you so much for joining me today. Matt. I think we’ve got a great topic here to dig into, one of our favorite things to fix up, which is dirty data. So I’m going to start with having you introduce yourself and how you come to data.
Matt: Oh, absolutely. It’s such a pleasure to be here. I’m really excited that you asked me to come on and it’s all things data. And, when we first met, it was really exciting to meet another person passionate about data also in the nonprofit space and just like serving this community that I think it’s overlooked a lot of times.
So, let me back up though. Hi, my name is Matt, Matt Bratton. I’m the founder of TMB analytics. And that is actually my side hustle. It’s just something that I do as a way to kind of like build a brand out in the social sphere, as far as like what I represent on LinkedIn and TikTok and YouTube, where I make content related to all things data analytics and a very strong focus on Excel, Microsoft Excel.
my day job though, I’m actually the CFO of a SaaS company. And what we do at Aplos, which is the company is a nonprofit accounting software. So this is fund accounting specific to the needs of nonprofits. And what that means is when you’ve got. Dollars that come in that are supposed to be earmarked for a very specific purpose through proper fund accounting, you’re able to generate an entire trial balance specific to that fund.
And you can see that across the board. And it’s it’s super valuable visibility. A lot of times it’s required in order to be good stewards of the dollars that you’re receiving. And so it’s a great company. It’s a great, great place to be. And I’ve had a lot of fun working there over the last 4 and a half years about.
Alexandra: Excellent. So you have lots of different places that you’ve got great experience with data, which is why it’s going to be such a great conversation today.
Matt: So fun.
Alexandra: Awesome. Well, one of the things I know that Excel, I feel like is one of those things that people love it or hate it, right? A lot of people that are super deep in the data space can be rather dismissive of Excel, right? That they say, Oh, it’s not a database. I mean, I’ve seen t shirts that say Excel is not a database.
Um, but I think from the nonprofit world, Excel gets overlooked because it’s a very, very inexpensive tool, right? Most of us run Windows in some capacity or Office, and so we have access to Excel already. and it has a very low entry point, right? You don’t have to know a lot to get started with it. So I do know that people have a lot of experience.
or have a lot of opportunity to be able to actually put Excel to some good use to get some important things out of data. And so, I know we’re going to talk specifically about certain aspects of some dirty data, but I’d love to also kind of weave in some basic tools and activities that people could do, you know, with tools that they already have sitting on their computer that maybe they’re overlooking.
but before we get into that, let’s talk a little bit about, contact lists, right? That’s going to be one of our. Topics of dirty data. so share with me some of your experience of like, what do you see the challenges around getting good clean data are and Why is that even important? Why should we worry about that?
Matt: Yeah, no, it’s a good question. And just to kind of reflect on some of the things you’ve said, there’s, irrational, passionate disdain in the data community, around Excel. And I like to think of it as just kind of like a, when you get to the point that we are with technology, you get people who are actually growing up through the education system that are coming out wanting to do.
Analytics, which just wasn’t a thing in the past. And I think it’s great, but what happens is it becomes such a very focused discipline that it’s, it gets detached from the business that it’s supposed to serve. That’s what I see a lot of. It’s you hear people talk about soft skills and business acumen, and then the purists will talk about like, well, I I’ve got my Python.
So I’m okay. And it’s just, look, the rest of the business, most of the business world does in some form or fashion run on Excel, like it or not. I don’t care what your t shirt says. It’s out there. It’s prevalent. And I hear the complaints that it is error prone and all of these things. It’s like, look, just because the tool has a low barrier to entry and the user can get in and do some damage, doesn’t mean that the tool is necessarily the problem here.
it’s more about the education and making sure that we know how to use it responsibly. And what are its strengths? What are its weaknesses? And where should we draw the line and say, Nope, this is no longer an Excel task. It is not a database, but we need to put this data over here. Right? And so, kind of getting back to the topic of, of, like, contactless and dirty data and why should we care? Let’s talk about, like, what dirty data even is, I think just to kind of, it just, it sounds so generic. I would say if you think in terms of we use the example of a contact list, that is like the lifeblood of a lot of nonprofits, right? You need that contact list. You need those donor lists. Those people who are have a vested interest in the success of your nonprofit or your cause.
To help address an initiative or a cause or a purpose or a why people believe in that. And then if you want to get their resources, you need to be able to contact them. So, what do you do? You need a contact list. A lot of times these contact lists, they live in some sort of an ERP or a very off the shelf system, like CRM. But what tends to happen is, How that data gets in there could be problematic and therefore how it comes out. This is classic garbage in garbage out becomes a problem because what good is the data in the system. If you can’t use it or leverage it. What if These are the most common things that I see, you know, names are jacked up salutations get in the way hyphenated names, middle names, 1st, middle last.
Addresses and keeping those up to date the way that people present their addresses, phone numbers, people just key in numbers, they may put parentheses, they may put dashes, a lot of it goes back to the source system and the collection process and making sure that if you see a trend or, oh, my gosh, my phone numbers are always messed up. How are your phone numbers getting in the system? So that’s a question that you need to ask. You need to think about stopping the bleeding, likely, before you go through some sort of a big cleanup effort. Because if you’re cleaning up while you’re going, you will never stop cleaning up. You need to start like kind of tracing things back to the source.
So how are we collecting phone numbers? Is it a form on our website that gets ported in? Are we manually doing this? Is it some combination of the things and setting rules and just being disciplined about executing to those rules to ensure that data that’s going in is going to be useful when it’s coming out and maybe phone numbers are a less good example, because a lot of times this goes down to, like.
Being able to mail people forms or, request for pledges and things like that. Those addresses are where the mucky muck really becomes a problem. And making sure that things are able to be sent. You’re not wasting money on postage that’s just going nowhere. You’re not sending it to the wrong people.
All of those things need to be considered. And it all goes back to data quality. I think that at the end of the day, that’s what it’s all about.
Alexandra: Yeah, no. And I love that you backed us up to like, what is even dirty data? Because I do think that that’s a term that gets thrown around a lot, but we’re like, what do we actually mean by that? You know, and I, I liked in your examples, you know, you talked about inconsistencies and I think that that’s like one of the most common places that dirty data, right?
Like that’s something that causes problems and data, um, is, is those inconsistencies. And as humans, Okay. We don’t care about inconsistencies because we can create. analogous connections. We know that a phone number separated by periods is the same as a phone number separated by hyphens. and so to us, that doesn’t seem different.
But we have to remember that one of the points of data is to store it in a way that can be read by a machine and processed consistently. And in order to do that, right, we’re going to need to make sure that we’re being consistent in a way that’s consistent to a machine and not just To us as humans, and that can be a hard step to take of like that consistency.
and so you mentioned like that. Sometimes it’s, human error, right? I’m keying in and I just key it in wrong, make mistakes. But also then as a human, I might do my phone numbers one way and you do your phone numbers a different way. And if there’s no culture around that, if there’s no training around that, if there’s no regulations around that, that can just continue.
And if you’re constantly trying to fix that at the end, you’re going to be spending an awful lot of time deleting parentheses and replacing periods with hyphens rather than just getting everyone to put phone numbers in. The same way at the beginning. so I think that’s, that’s a great point. but some of the other problems with, dirty data may be a little bit harder to identify The source of it, right?
Like some inaccuracies. You talked a lot about accurate addresses, right? And I may be writing down an address exactly as someone gives it to me, but, either, maybe there’s something that’s, that’s necessary. They, they forget to give me their apartment number or, or they just say, they give me the street address and the building number, but not, where the mail actually needs to go or whatever it might be, right?
There might be things that. As a human, I look at the address and it seems fine. How do I work with those sorts of dirty data elements?
Matt: yeah, I kind of have a weird, maybe it’s not weird, but I think about a lot of these things very spatially. Like, when I think about an address, you think about what are the different components that make up an address and a lot of times when I’m being forced to clean data, because, you know, we work with a lot of nonprofits and a lot of times we’re changing over from 1 system to another and you’re moving data from an old system where there weren’t rules and regulations and we’re trying to stand up rules and regulations.
you have to deal with the consequences of your previous actions, which is cleaning this stuff up, right? So what you do is you almost solve for, there’s probably people out there that are gonna cringe, but you solve for the worst case scenario. Where, what, how complex could an address get? Right? You’ve got a number, maybe you’ve got a, a ordinal coordinate or whatever.
North, southeast west, you’ve got a, a name. Then you’ve got, I don’t know what to call it, an avenue, a street or a this or a that. And then you may have. a floor or a suite or this, it’s really understanding what are we collecting and what are the possible use cases of it. Therefore, we can accommodate to that and ensure that we are going to capture for the most part, any possible combination of things that could come in.
One of the problems I see is a lot of companies, they get so narrow in their focus. It’s like, All we allow is like street name, zip code, something like that. And then it just free form for people to put in whatever the heck they want. And it just causes all kinds of mucky muck. What that does is forces them to manage to the exceptions, but you’ve effectively enforced all of these exceptions.
Because everything doesn’t fit into that very fine box. Therefore, anything that doesn’t, which is most things is an exception. So you have to manage to that what you need to flip it so that you’re managing to the norm, or you’re managing to what the standards should be. And then, in the event of exceptions, you can deal with those one off and there’s far fewer of them because you’ve taken into consideration what could be.
Upfront it’s it’s putting in that work up front. I love that. You talked about like, consistency. There’s in my past life, and I try to bring it forward. Every company I’ve been at. The concept of data, consistency and integrity, and to the point of putting together groups of people who it’s like, this is our purpose.
Like, we are focused on data, consistency and integrity and making sure that this is a topic that is 1 of the least exciting topics to talk about. But it is critical. To our ability to execute in whatever it is that we’re doing. So really staying focused on how can we be consistent? How do we maintain integrity?
And therefore, ultimately, the integrity and consistency of the data and how we capture it.
Alexandra: I think that those, those twin concepts of consistency and integrity are great because the consistency has to go into, like I said, like how we collect things. So are we collecting full first names? Are we collecting preferred nicknames? You know, that’s a consistency thing. because Calling me Alex versus Alexander, both are correct, but it may mean something different in the system, right, in terms of what I’m trying to match to or what we’re trying to use that field for.
Where’s your integrity? Now you talk about, that’s like the accuracy of the data, like, is the address actually going to get a piece of mail there? That’s the integrity of like it being correct, not just consistent, because that could be consistently wrong. I could, I could collect it exactly the same way every time, but it’s still wrong.
Matt: Absolutely. And it happens all the time. I love that you brought up the, too, the, the naming convention. That, that’s another thing that I see is you’ll have a, at best, first, middle, last opportunity for people to key things in. And what do you do when there’s a preferred name like.
My name is Thomas Matthew Bratton, but I go by Matt. So like thinking about those sorts of things and making sure that you have that, like, is there a preferred name and maybe you defaulted to their first name? I don’t know. But making sure that you’re collecting all of those possible uses. And considering it and implementing it so that it does not become a problem down the road.
Alexandra: And I think that that’s an oftentimes missed piece of data integrity, is it’s not just about being accurate, it’s about being usable for what you’re trying to do. So, right, if I am trying to, Get somebody government benefits. I need their full legal name, right? If my nonprofit is helping facilitate Medicare or Medicaid or whatever, I need to know that it’s Thomas Matthew.
Matt: Yeah,
Alexandra: But if I’m going to mail you an appeal letter. You’re not going to really like it when it says, Dear Thomas Matthew, you’re going to be like, yeah, you don’t know who I am, right?
Check it out. And so in that case, I would need that preferred name. And so I think, you know, you bring up a really good point of, and I liked you, you mentioned this about the addresses of getting clear of like, what are the discrete elements that I need?
What are all of the possible discrete? Elements that I need and then which of these are the norm. So I’m going to really make sure that my system’s engineered to catch that. And then which of those might be exceptions and I can have a place to handle those exceptions. and I think that, you know, it ties into the that name thing again, like, there’s a lot of different places where this could be the case that, you know, if I, because I see this like with, um.
When addresses are designed to capture US addresses,
Matt: Oh, yeah.
Alexandra: right? And it’s like, well, as soon as right, as soon as I’m trying to put in a UK address, and it’s asking me for a state, I’m like, what do I put in there, right? Like, I guess I could put the county of the UK that and so again, like, you can, if you’re not thinking about, What’s going to come in and making it appropriate to capture that you could really get yourself stuck in terms of, of maintaining both that consistency and integrity, because you haven’t thought about the components you’re going to collect and you haven’t thought about how you’re going to use those components down the road.
Matt: and I think this also, I think it would be probably prudent to bring up the idea that there is a point of overengineering and that gets back to managing to exceptions, right? If maybe you’ve got an entity and. 1 time, somebody wanted to, they’re like, I’m visiting from out of country, but I want to do this thing.
And, oh, my God, we got to re, engineer the system to capture international people that, you know, maybe you need to take a step back and think about, like, is this a going concern? Yes or no, making sure that you’re not managing to that exception. But if it’s part of your business. Absolutely, make the effort, take the step back.
Think through how this should look in an ideal situation for what we are trying to accomplish. Then you can move forward.
Alexandra: Yeah.
Matt: Good call out there.
Alexandra: No, and that’s a great point. That’s that like balance of saying, what’s the 80 20, right? What’s the 80% that I can make sure works perfectly. And we’ll just understand that there’s going to be a certain component. That’s not because in order we’re going to screw up the 80% if we try to make it work for the 20%, which doesn’t make sense to do that.
I am curious in terms of. so you know, you talked a lot about like, first is stop the bleed, right? So we’re going to make sure that we’re engineering these systems that are capturing data. We’re going to think about what do we actually need to capture? What needs to go in that part of it’s the tech side, right?
Let’s make sure we have a field for preferred name, but part of it’s also training,
right? Making sure that now we’re going to educate everybody. Full name goes here. Preferred name goes here. Please don’t put parentheses with their preferred name in the full name field, right? There’s that education piece, but let’s say you’ve done that.
But now, like you said, we’ve got the historical data that we’re stuck with or data, we purchase a list and now I’ve got to figure out how to like merge this purchased address list into our nice clean managed list. How do we start dealing with that actual cleanup step?
Matt: Yeah, this is a situation where there’s going to be a lot of flavors of this. There’s people who are experts in this who will go about it 1 way. I’m going to go about it. Probably a different way. And then there’s, this is a spectrum and I don’t know that there’s a right, wrong or otherwise it should be taken on a case by case basis.
Like, where are we starting? Like, what is what is our ideal? Where are we going? Like, what are we trying to achieve and what did we just get? And what about this thing that we just got? Is good, bad or otherwise, I think 1 of the things that people tend to do is get wildly overwhelmed when they get something and it’s like, oh, my God, this is 20, 000 rows and look at that.
It is all the number and the address and the, the city and state, or they’re all combined or, you know, this weird CSV file with, uh, you know, it’s all just basically a text block. What do I do? We don’t stop to really think about like, look, we’re going to break this up into, into chunks. But we need to know where we’re headed.
A lot of times people just jump in, want to start cleaning and just start, like, trying to make sense of the thing. It’s like, that’s fine from an optical standpoint, but don’t break anything yet. Figure out where you’re headed and then sort of get your bearings, do your research in the thing before you move on.
There are different tools. There are different softwares. You could use different, like, coding languages to probably accomplish some of these things. If you’re operating in Excel, which a lot of times I am because that’s where I’m comfortable. That is, what is it? I see numbers from 700 million to over a billion users of Excel.
it’s there and you can use it. And even if you’re over a million rows, like people tend to think is the limit, you could use power query and automate some of the cleaning. It really just depends on what are you trying to achieve? And what are the inherent issues that you’ve observed in the data? Is it strictly formatting?
Is it just the way that it is handed to you? Then that’s probably an easy fix. Is it actually like messed up data? Like there’s all sorts of special characters or something didn’t come out of the source system very well. Those are going to take a different type of approach. So it’s really about, it’s not dividing and conquering.
It’s getting very explicit on what needs to be done and where are we starting so that we can kind of bridge the gap and figure out how to attack this beast. Because a lot of times it is a Big project. There’s a lot of moving, or a lot of potential moving pieces, and you want to make sure you’re moving the right ones.
Alexandra: That’s a great point in terms of there may be lots of things that you could get involved in, you know, you just get this new mailing list or you just get these, new contacts and we have to figure out what to do with them. And you may see that there’s like this free notes field that’s got all sorts of stuff in it.
And you’re like, how am I going to make any sense of that? But if you stopped and we’re like, what am I actually going to use this for? You’d find out that you don’t even need that field. So why worry about what’s in it? Or why worry that some of the characters are getting dropped out? Like. I don’t need that field because here’s what I’m actually trying to do with it.
So I can set that aside and not worry about it. that resonates so much with me because I do come back to that with nearly every data problem. It’s like, hang on, where are we trying to go? Like, let’s get real clear with that first before we do anything else, because it’s going to be what we measure our choices against.
And then, you know, that you then said, okay, so if here’s where we’re trying to go, where are we starting from? What’s the actual situation here? How far are we from where we’re trying to go? And then that highlights for you, like, okay, well, if what I was trying to do was use this list to mail people, you know, a certain, let’s say, like, appeal letter, or I’m trying to invite them to a program, I can identify, okay, what’s necessary to do that thing, and can my data do it now, or are there changes that need to be made to the data in order to To get it there.
That’s
Matt: Exactly, yeah, no, and I think that’s a really good way to think about it as well on a use case basis or like, what, what is the objective? It kind of keeps coming back to that while you’re talking. I’m thinking there’s, there’s a couple of quotes about elephants. the 1st, 1 is like, how do you eat an elephant?
1 bite at a time? Like, that’s always something that’s good to keep in mind when you’re dealing with these large, you know, big, hairy projects. There’s another 1 that I haven’t heard a lot of other people say, but it’s one that I heard and I really liked, which is like, how do you sculpt an elephant, using marble or whatever it is.
And the answer is like, get a piece of marble and remove everything that doesn’t look like an elephant. So, uh, and you could use any example there, but it’s like, okay, you start with this big, just slab of data. But like, what are you trying to get? And a lot of times it’s not even the cleaning, it’s the removing of excess.
And like, Oh, my God, there’s like 700 columns and this and that, and I don’t know what to do. It’s like, right, but you only need four. So let’s get started there. Let’s isolate the four that you need, and then let’s reevaluate. And it’s, it’s taking that, that step by step process, like slowly eating the elephant, chipping away the things that don’t need to be there, and then eventually it will reveal itself.
Alexandra: a great point. It is so easy. And I fall into this trap a lot because I’m always like all the data. I want all the data. Um, but to remember, right, remember that actually getting rid of data can make your life so much easier can making so much. Simpler to handle. and so by focusing on just the parts you need, like you said, getting rid of the stuff that you don’t like, let it go, put it somewhere else.
and so I think that’s a great analogy. I love that. Just take everything away. That’s that’s not the elephant is perfect. one of the other things I thought that was interesting that you brought up as well was like the idea of. What kind of challenge are we trying to solve? I mean, like, are they formatting problems?
Are there actual just inaccuracies in there? Because one of the things that I realized as you were talking is that, like, some of those things are very easy to solve in a tool like Excel, right? Formatting, you can, there’s a lot of great formulas that can help you change periods into dashes for phone numbers or whatever it might be.
But there could be things like inaccuracies where you’re going along and you, it requires a human review, right, to be able to interpret what’s in there to be like, oh, I have to fix that because they’ve done blank wrong, you know, and you may be able to identify patterns in there. Sometimes there are patterns where then you could figure out a formula that would help you, but sometimes it just requires somebody who actually knows what’s going on.
To like go through it and say, Oh, they were signed up for the wrong program or whatever, you know, and you have to fix, fix it with human review. And I think breaking that down into understanding, like, what are things that I can use computers to fix? What are patterns that are consistent that I could define this versus what is like an inaccuracy that’s going to require human intervention?
Because I think sometimes we use humans to do the computer stuff, which we’re terrible at. And then we try to get computers to do the human stuff, which they’re terrible at. And so identifying those sort of those two broad categories of fixing that has to happen is important from the beginning so that you you head down the right path and using the right tools.
Matt: yeah, no, I that’s great. And I, very much agree. I think there’s, there’s different types of people who, when they encounter kind of dirty data in the wild, they react in 1 of those ways, which is like, oh, my God, there’s got to be a formula to fix all of this. Sometimes there isn’t. I hate to break it to you. Chat GPT may or may not be able to help you out of this one, right? It does need a human. Review, but through that process, like, maybe you only need to invest 10 minutes to looking at the things and going like, oh. There is a pattern. Good point. now that I understand the pattern, I can isolate these things and we can carve off that chunk.
And now we’re cooking with fire. So I think that being hesitant to get your hands dirty or accepting of the idea that. Yes, you may actually have to do some work to review this stuff. That’s where a lot of people get hung up. I like to take it from the approach of, like, let’s get our hands dirty.
Make sure that we know what we’re messing with and then keep doing the 80 20 thing. It’s like, okay, I think 80% of these things we can handle like this. Boom, done. Feel good. Now this 20%. What’s the lift involved to fix the 20%? Are they purely manual? If so, is it worth it? Like, what are we trying to achieve?
what is the problem we’re trying to solve? And then deciding how to move forward. Sometimes that last 20% just requires a little bit of additional effort. And then that creates a situation where you can become efficient again, and then mop up another 80%. And you just get it down to the point where now you’re dealing with just a few exceptions.
And those just need to be handled on a case by case basis and. Accepting that this is. Part of the process is where a lot of people get tripped up because they think that, man, I shouldn’t have to be doing this much work. It’s like, well, maybe you’re right, but you’ve got to put in a little bit of effort to determine that.
And have, if you’ve done that, then great. If you haven’t then. Get to work.
Alexandra: no, I know. I’m curious if you’ve got a few favorite Excel formulas, that you find are the most frequently used around like cleaning contact lists.
Matt: Oh boy. Yeah. So there’s a few that are newer that I think have been kind of rolled out in more recent history that I, that have created some pretty cool things where I think some of the classics. So number one, I got to give it up for the trim function. Right? There’s. The number of times that you may encounter indexes that don’t match or other things like that, where they look identical as far as you can tell all the text is the same, but they just aren’t picking up as a match.
Because there’s like a hanging space at the beginning, middle or end that you just, you can’t see it because it doesn’t stand out using the trim function will help take care of a lot of those and promote consistency. So that’s certainly 1. historically, I would have said, you know, left mid and right functions to, like, grab.
Left number of this character. So like if you’re dealing with zip codes and you want to be able to break off that, for bunch in the back instead of the first five or something, it’s presented in a certain way you could do a right five, grab the text and you’re getting that last bucket. And then you can do a mid by counting and find so find itself is another good one.
So, find left right mid. Now we’ve got like text before text after text join. There’s a lot of these new functions that are, they’re just a little bit easier to not only understand what they’re doing, like, they’ve really worked on the syntax part on. Like kudos, I guess, to the Excel, developers, people who are like trying to make it a better, more user friendly tool.
So you’ve got like text before, that’s exactly what it says. It’s like, you’re going to grab text before a certain thing. So tell me what the thing is you want to find. Is it a space? Is it a hyphen? Is it a parenthesis? Whatever it is, text before, text after, does the exact same thing, just after. Text join is if maybe you do already have a string and you want to create like an address block, you can create a common delimiter along a text string and just say, I want to join this, this, and this, and I want to separate it by a comma or like a special character to create a space down or something like that.
There’s those sorts of things I think are, you can go a long way with just a few of those functions, assuming we’re not like dabbling in power query, which could take all of this to a completely different level for sure. But just quick and dirty work, you can go a long way with a lot of those,
Alexandra: Yeah, and I think that this is why, and it’s interesting because I’ve had a few people ask me, like, okay, if I want to do data in nonprofits, like, what do I need to learn? What tools should I learn if I’m brand new to this space? and I think, you know, you bring up such a great point that there is a lot you can do just learning.
Like a couple of, important functions in Excel will get you to a place where you can make a lot of use of the data that you’ve already got. Um, and so I think if you’re looking at just sort of taking that next little step, learning how to write a few basic functions in Excel, I do think is a really great.
Great first step. And when the time comes, you can learn Power Query, you can learn SQL, you can learn some of these other codes out there that let you do much more complicated things. but there’s nothing wrong with getting started with those smaller steps that still might yield some really great results for you.
Matt: Yeah, I agree with that. Bigly. I think that there’s this, I kind of started off by talking about there’s a lot of like, irrational, just like hatred towards Excel. That just, it doesn’t make sense, but it’s there and it’s. It’s kind of funny, I guess, if you think about it, but like, I’ve seen comments recently like, Oh, it’s just the kiddie pool of analytics.
Like, no, I think of it more as like a gateway drug. Maybe that I shouldn’t think about it like that, but it’s like a gateway tool for people to get exposure in a low risk, open playground to like start playing around and believe it or not, like it or not, you can go real far with solid Excel skills.
no joke, because The closer you get to the operations of the business, the more likely it is that they’re, they’re working in a lot of like ad hoc type requests where they’re dealing with quick analytical asks like, hey, what would happen if we did this? And I need a model for that. I’m sorry, but you’re probably not going to, you know, go into Python and build this thing.
you’re 12 minutes, right? That’s just the way it’s going to work. And then you can hand it back to the user. so it has so much applicability, but it also teaches basic logic and, ordering in different syntax that if you understand the things that you’re trying to do, once you get into the other types of coding languages, you’re like, oh, that’s just like doing this, or it’s just like, oh, it’s like doing a pivot table, and if I want to do this, then I have to do that or working in the data model.
So it feels like table stakes to me as a starting point, and it should not be looked out upon. I think that it’s. a very versatile tool.
Alexandra: I think it’s, like people being disparaging about using bicycles because they’re like, well, how are you going to get from New York to California? You’re like, well, if that’s what you’re trying to do with a bike, yeah, that’s going to be hard for you. But if you’re, if I’m just trying to get to the grocery store every day, a bike’s a great option.
I don’t need a driver’s license, I don’t have to insure it, like it’s a great entry point to get me moving.
Matt: no. And, and I see this, like I’ve been involved in martial arts for most of my life and you’ve got like people in mixed martial arts or people you’ve got like, Oh, this top boxer, what would happen if they fought like this, top MMA person or something like that? And like, yeah, but what would happen if you tied one arm behind their back and you, you gave them this, it’s like, come on now you’re just making stuff up.
You’re just coming up with an arbitrary scenario. To fit whatever thing it is that you’re trying to do to make yourself feel better. It’s like, look, this isn’t, us versus them. This is a, what are we trying to achieve? And what’s the best way to get there? And this is one way to get there.
Let’s just be okay with that.
Alexandra: Yes. and it’s like an example of like, don’t use it to manage where your flight teams are if you’re running like a multi international airline, right? Like, again, that’s not an appropriate use of Excel and it does come crashing down.
Matt: Excel is not a database. Like we’ve already, we’ve already established that. Right. So, yeah, exactly.
Alexandra: I love that. Well, so to wrap up, I’m wondering, do you have a good resource if somebody’s listening and they’re like, you know what?
I am inspired. I’m going to learn a few Excel formulas and like, take my first couple of steps. Like, do you got a good place to send them so that they can get started with cleaning that dirty contact list? Um, and using Excel in potentially a slightly new way.
Matt: Oh, my goodness. So one place that I might ask you to check out would be one of my profile. So I’m, I’m on YouTube. I’m on tick tock. I’m on LinkedIn. And I do cover a lot of Excel content. So it’s just at Matt Bratton on all of those platforms. It’s something that I’m passionate about, and I have started to really get down and dirty on Excel.
I’ve got in a newsletter that I’ve got to actually 1 that’s specific to analytics careers called the daily analyst, and I’ve got 1 that’s specific to Microsoft Excel called the data Excel daily. Maybe I need to merge those at some point because it’s very similar type of topic where it’s like I want to make analytics accessible and highlight just how prevalent it is within the workforce and how valuable just.
I’ll call it critical thinking skills are just another way of saying analytics and then Excel being the most accessible tool, being able to wield that in a responsible way and use it sort of to start exploration and, getting getting into this, area of work. So check out those things. get on the newsletter.
I’m always sharing tips and other things. I also on YouTube have a 4 part Excel for analytics project series. That’s. Go I would say if anything, watch video number one because it gets into some very basic tips, tricks and other things for leveling up your Excel. And if you stop there, then you probably already got all of the things that you just said is like some very basics.
And then the second video gets into like data cleaning and other things. So. All good resources. And then by the way, there’s just tons of awesome content out there in general. no shortage of it. You don’t have to just limit yourself to me.
Alexandra: I love it. Well, we’ll link to all of those things in the show notes. Um, so everyone can find those and hopefully, like I said, sort of take their, first or next step, in Excel to help those contact lists and all those other things that we’re using. Like you said, the ad hoc questions, that quick, like, Hey, I just need a summary of something or account of something or an average of something, um, that are things that we can do just with a real quick, You know, entry into into Excel.
So I think that that’s a great thing for people to be comfortable doing regardless of what your role is in an organization.
Matt: Absolutely. Yep. That’d be great.
Alexandra: Awesome. Thank you so much for your time today.
Matt: Thanks for having me. It’s been a, been a joy.
You have been listening to Heart, soul, and Data. This podcast is brought to you by Moroccans, an analytics education, consulting, and data services company devoted to helping nonprofits and social enterprises amplify their impacts and drive through data. You can learn more at Moroccans Dot. M E R a k i n o s.com.
I need you to create provide a summary of what the episode is about. Use the entire transcript as your reference. It should appeal to an audience of those interested in data literacy and data analytics. Here are some examples from previous episodes. Use the same style:
- In this episode, we sat down with Rebecca Gosla of Simplicity Education to discuss what it takes to succeed as a data manager, how being a data manager can mean different things to different orgs, what the day to day experience of a data manager looks like, and what those of us who are not data managers can do to better support this role so that we all have the information that we need to be successful at the change we are trying to achieve.
- “When you’re thinking about doing a research study, define and design. So you define what you wanna know, then you design how you’re gonna find that out. That’s everything. Everything goes back to those two words.” If you’re one of those people who think that scientific research is just for academic scientists or the lab rats working hard at their lab bench benches with their pipettes, today’s episode is for you. I am joined by Neil Krohn, founder and principal of Advanced Healthcare Analytics, to share his expertise in doing research that really matters, and how research isn’t just for academic scientists. Stick around till the very end of this episode and learn how your organization, even a small nonprofit, could benefit from designing your own research projects.
- Think about the pieces of technology in use in your organization or your team today. In most nonprofits out there, you’d be surprised that there are dozens and even up to 50 different pieces of technology being used across your organization. In today’s episode, I am joined by Tim Lockie of The Human Stack, who specializes in optimizing this interface between technology and humans. Learn how these tech systems work within the ecosystem, and how a CIO plays a part in managing these interactions—regardless of how big or small your organization is. We also discuss the skills required for a chief information officer (CIO) position, the need for effective communication and collaboration within organizations to harness the power of data, the challenges nonprofits face in utilizing data effectively, and how you can take action to address these challenges
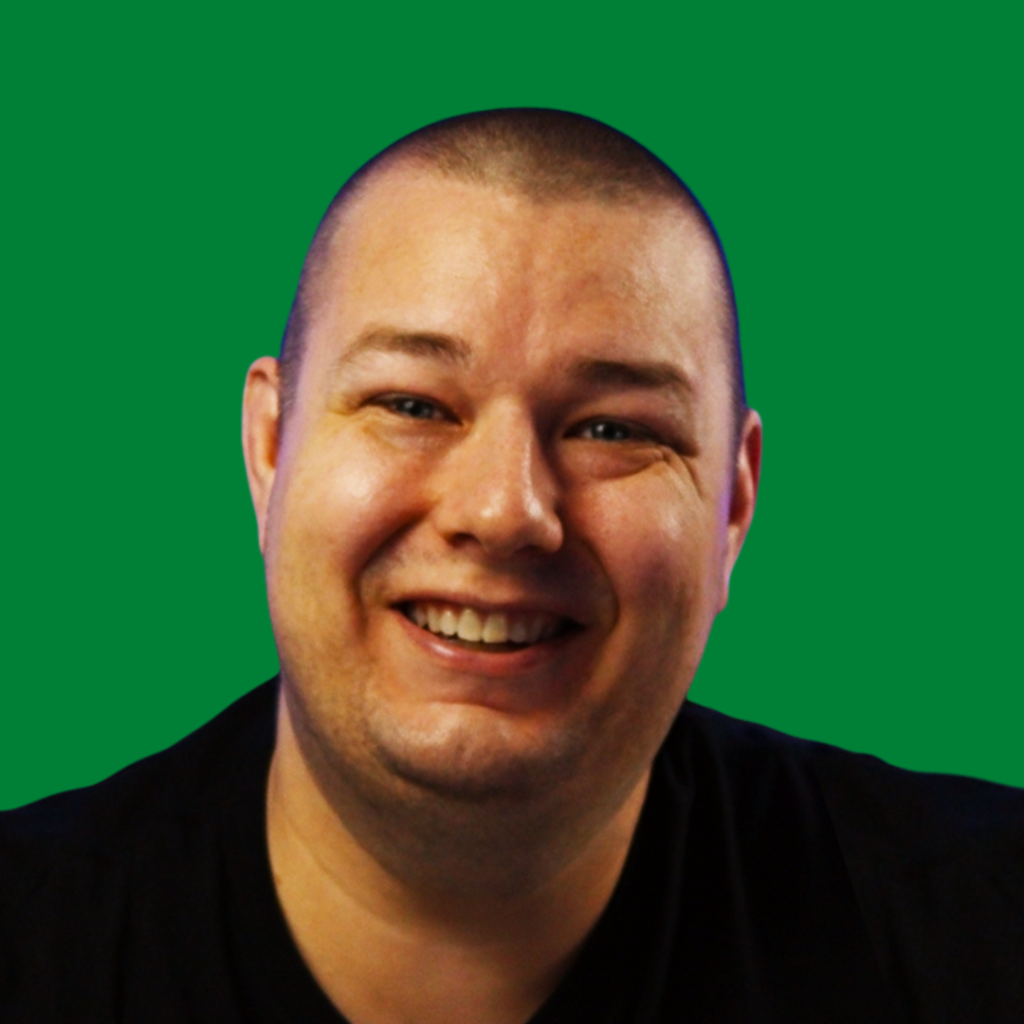
Matt Brattin
Matt Brattin is a SaaS CFO, data nerd, and Excel fanatic who uses his platform to help professionals “doing the work” learn new ways of thinking and doing that will propel them in their careers. As a nearly 20-year professional in the space, he’s looking to support the development of the next generation of data leaders.
Comments are closed